To Shift Opinions in Online Conversations, Start by Building Trust
Debates on social media follow a predictable pattern: opposing sides exchange a few insults and double down on their original positions before retreating to separate echo chambers. New research from Yale SOM’s Tauhid Zaman suggests that starting by establishing common ground makes it possible to make connections and even change some minds.
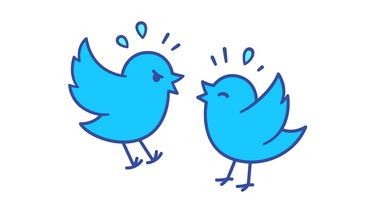
Like many of us, Tauhid Zaman, an associate professor of operations management at Yale SOM, has noticed that social networks offer an ideal habitat for the proliferation of strong opinions—but maybe less so for genuine discourse.
Over the past few years, Zaman has built several research projects around the study of online opining. He has looked into whether artificial intelligence can help pinpoint ISIS affiliates and other social media actors propagating dangerous messages and how to identify bot networks trying to skew public opinion. In a new paper, he investigates how users posting strong (or even extreme) opinions on Twitter respond to attempts at persuasion.
“The first part of my research in this area was about finding these extremist viewpoints or the bots trying to manipulate opinions—and just identify that there’s a problem,” says Zaman. “After detecting those opinion shifts, the next natural question is, ‘If they’re moving that way, could I move them the other way?’”
Zaman intuited from time spent on Twitter that users’ most frequent approach to persuasion on the platform—“trying to do hot takes, arguing with people, dunking on them”—seemed not only ineffective, but probably just left all sides more entrenched in their original positions.
Zaman says online opinions can be shifted by “pacing and leading”: “I come close to you” by professing similar opinions “and then I take a step. I start to pace you and lead you somewhere else.”
This impression was strengthened as he dug into others’ research on persuasion over online networks, including a 2018 paper that found both Democrats and Republicans doubled down on their own political viewpoints after regularly reading Tweets from the other side—a pattern widely referred to as the “backfire effect.”
In Zaman’s new research, conducted alongside Qi Yang and Khizar Qureshi, he tests the effectiveness of a different approach to online persuasion—one that begins with an attempt to build trust over time. He calls the tactic “pacing and leading”: “I come close to you”—that is, by professing similar opinions—“and then I take a step. I start to pace you and lead you somewhere else,” Zaman explains.
Zaman’s findings indicate that the pacing-and-leading approach was successful in nudging real Twitter users with strong anti-immigration sentiments to use less extreme language over the course of a few months. The persuasion effect was strongest when pacing and leading was combined with a Twitter-specific form of contact—liking subjects’ tweets. On the other hand, Zaman finds that exposing anti-immigration-minded Twitter users to a stream of pro-immigration tweets over the same period only intensified the potency of their anti-immigration language—seemingly a sign that the backfire effect had been triggered.
Zaman believes that public health is one arena where the pacing-and-leading model should inform public messaging strategies—especially when it comes to persuading people to get vaccinated against COVID-19.
“I think public officials’ approach to persuasion about the vaccine has been totally misguided,” he says. “There are people who don’t want to get the vaccine, and the response has been to tell them it’s good and jam that message down their throats. That’s just going to create more antagonism, more skepticism, more denialism. Persuasion takes time; it’s not instantaneous.”
In Zaman’s study, the time allotted to try to shift opinions was about five months (late September 2018 to early March 2019), a period broken into five phases. During phase zero, the researchers created three bots—that is, automated Twitter accounts—to enact various persuasion methods. One bot was simply a control; it never posted content or interacted with subjects. The second bot employed what the researchers termed the “arguing” method: consistently posting strong pro-immigration content. The third was the pacing-and-leading bot, which initially tweeted anti-immigration sentiments and gradually became more pro-immigration.
The researchers’ subjects were real Twitter users initially gathered by searching for anti-immigration hashtags like “#RefugeesNotWelcome” and “#BanMuslims.” For the experiment to work, a portion of these users would need to follow the bots, so that the bots’ tweets would appear in their feeds. To get followers, the researchers randomly assigned bots to the usernames they’d gathered; the bots then liked a recent tweet of each assigned user and followed them. About 19% of the subjects, totalling 1,300 users, responded by following the bots.
In phases one, two, and three—each of which lasted about one month—the arguing bot posted a pro-immigration tweet once a day. Though the pacing-and-leading bot tweeted at the same frequency, its content shifted over time—from anti-immigration messages in the first phase, to more uncertainty and concessions of pro-immigration points in the second, to a stream of tweets in the third phase that were as pro-immigration as those of the arguing bot. In the fourth phase, all bots were quiet, and the researchers measured any lasting effects they had.
In addition to the variation in messaging, the researchers also tested how subjects responded to interaction from the bots—that is, the bots liking their tweets. “This interaction can serve as a form of social contact in an online setting and potentially lead to more effective persuasion,” they write. To investigate whether this kind of engagement was effective, they randomly assigned half of the subjects in the arguing group and half of those in the pacing-and-leading group to the contact treatment, meaning their tweets occasionally got a “like” from the bots.
To track how anti-immigration sentiment shifted (or didn’t) in users’ tweets over time, the researchers zeroed in on the prevalence of one word: “illegals.”
“We saw that if subjects are using that word, they were using it in an aggressive way,” Zaman says. “It was the most straightforward signal that the language was toxic.”
By plotting usage frequency of the word “illegals” across phases and treatment groups, the researchers found that combining the pacing-and-leading tactic with the contact treatment appeared to have the greatest persuasive impact—especially in phase two, when the pacing-and-leading bot was softening its stance and expressing uncertainty. Conversely, for the group assigned to the arguing bot and the contact treatment, the second phase spurred the highest frequency of the word “illegals”—seeming to indicate the presence of the backfire effect.
Zaman acknowledges that the study leaves open important questions, which he hopes he or others will pursue: “Do we need that first phase? Perhaps if my opinions are already close enough to yours, I don’t need it,” he says. “And do we need the third phase? Maybe it’s possible to stay nuanced and that’s enough to get the job done.”
Another open question: can the pacing-and-leading approach have a symmetric effect both ways? In other words, could it be used to pull opinions into more extremist territory? Though the experiment doesn’t answer that question, Zaman suspects that it’s possible—and that, for this reason, the pacing-and-leading tactics should be handled carefully.
“I would say that this tool is very powerful,” he explains. “If you use this thing the wrong way, you could take a moderate person and radicalize them. This has to be used by policymakers who have good intentions and know what they're doing.”