Can We Protect Our Election from the Bots?
In the wake of the 2016 election, Twitter found that more than 50,000 Russia-linked bots were active on its network during the election, reaching more than 1.4 million Americans. What should governments and social media platforms be doing to detect and counter bots as another presidential election approaches? We talked with Prof. Tauhid Zaman, who has carried out a series of studies identifying bot networks and assessing their impact.
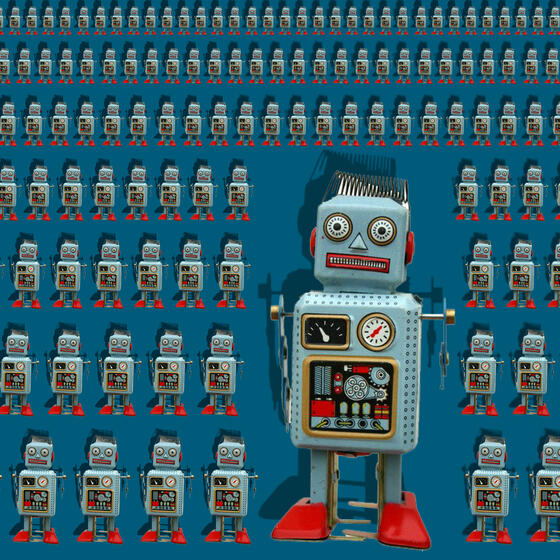
What’s a Twitter bot?
As we define it, it’s one person is controlling multiple accounts to amplify their voice. Usually these accounts are automated in that the person doesn’t actually click the tweet button or the retweet button. There’s code that detects tweets and retweets them automatically. These things are running 24 hours a day, so they seem more active than an actual human being.
And what they do is amplify certain kinds of content; what they amplify depends on what their goal is. If you want to amplify, let’s say a pro-Trump message, you’ll retweet anything by Trump or any of the MAGA people. If you’re a pro-Biden person, you create a bot that amplifies any tweets by Joe Biden or a former Obama official praising Joe Biden, things like that. The bot’s goal is to basically make it seem like there are more people talking about a topic than there actually are.
How prevalent are bots on Twitter?
In analyses I’ve done in the past, I’ve looked at how many of the participants in a discussion on a particular topic are bots as identified by our algorithm. It can be as low as 1%. I’ve seen as high as 7% or 8% for some topics.
In the protests against Macron in Paris called gilets jaunes, yellow vests, we were seeing that 10% were bots on Twitter. That’s a huge number of bots. We also saw 10% bots in the Brexit debate. Which is thousands of accounts in raw numbers.
What’s dangerous about bots?
“If you are advocating for an issue, and not many people are talking about it, you can use the bots to create the illusion that there is traction.”
The danger of bots, or maybe the strength of the bots, depending on how you view it, is that if you are advocating for an issue, and not many people are talking about your issue, you can use the bots to create the illusion that there is traction on the issue. When the bots are effective, you’ll see a topic trending on Twitter. Trending is supposed to be when a lot of people, organically, start talking about a topic because it’s interesting. But if you have enough bots and you’re clever about it, you can game the system.
Sometimes at 5 a.m. or 6 a.m. you’ll see things trending that are critical of Democrats. People suggest that may be because it’s the beginning of the workday in Russia, and Russian bots are amplifying some anti-Democratic hashtags.
If you want to look at a higher level strategy, let’s say that I was trying to interfere in the U.S. election, and my goal is to help Biden. I would just amplify and retweet anything saying coronavirus is terrible. People are dying; there’s deaths everywhere. I would amplify that story in networks where the voters are of value to me—Pennsylvania, Ohio, Michigan. Find people in those regions, get them to follow me, and then retweet things about coronavirus being a disaster and how the government’s failed in the response and it’s just a mess. If I was going help Trump win the election, I would find stories saying Biden opposed fracking and amplify those stories in networks of Pennsylvanians, where fracking is a key issue.
What are the challenges in identifying a bot?
They evolve. The first generation of bots are created, and the social media platforms find ways to detect and shut them down. Then people get smarter by making better bots that avoid detection, until they detect those bots. It’s an arms race.
Two or three years ago, we built an algorithm to look for bots. We figured that the way bots behave is they just retweet stuff. So a bot was easily found because it’s retweeting a lot of people and no one’s really retweeting it. That was a signal that this is probably a bot. You could look at a whole network of people talking about a singular topic, and you can see who the bots are because their behaviors are anomalous.
Today, a lot of the bots are still of that nature. But the new thing is when you have an account that someone is actually tweeting but that one person has multiple accounts. That’s more effort. It’s not automated, but it’s still artificial. And that makes it harder to tell if it’s fake or not fake
Could I be interacting with bots on Twitter and not know it?
You might be. There are chatbots being developed by Facebook and Microsoft and many companies that talk to you when you’re buying an airline ticket or something. It’s in its infancy phase now, but the technology exists. My group is thinking about trying to apply some of the AI tech out there today and see if we can generate original tweets for a bot. Just tell it, “Hey, tweet something that’s 70% pro Trump or anti-Trump,” and then it generates a tweet of that sentiment. This type of controlled text generation has made some exciting major advances recently, and I think in a few years you’ll be talking to a bot and won’t even know it.
In your research, you found some aspects of what bots have in common with each other. Can you talk about what you found?
We had human beings go through thousands of Twitter accounts and do an-in depth human analysis to say, based upon your best judgment, is this a bot or a human? That’s the most reliable method we have right now. After a lot of intensive labor, they label a bunch of accounts as being bots or humans. That was our training data. And then we trained an algorithm to look at that data and match those patterns.
What we found was that, as I mentioned, the bots retweet a lot of people. They don’t retweet each other. The bots don’t talk to each other and no humans retweet the bots. So if you find a set of nodes in the network that are acting that way, those are probably a group of bots.
Previously, bot detectors would take one individual account, look at its behavioral profile, and try to guess if it’s a bot or a human from its individual behavior. Our advancement was to find a gang of bots simultaneously. Looking at the whole network you can identify more easily and more accurately who the bots are based upon the group behavior they exhibit.
Do bots actually change people’s opinions?
Our technique to assess how much impact the bots have on discussions is based on three factors: connectivity, activity, and opinion. For a bot to have impact, it has to be connected to the network, meaning it has followers. The bot must be active to have impact, meaning it is tweeting. Finally, to move opinions, the people hearing the bot must not already agree with the bot. Our assessment technique combines these three factors to give a measure of the bot impact.
“With Brexit, the bots didn’t really have an impact on opinions in the network. They were getting drowned out by everybody else talking. But with the gilets jaunes protests in France, they had a huge impact.”
In our previous research, we studied Brexit and the gilets jaunes protest in Paris. In Brexit, the bots weren’t having much of an effect on the aggregate opinion, but with gilets jaunes, they’re having a big impact in the Twitter discussion. The gilets jaunes network was several tens of thousands of people, and I think about 10% of that network of people was bots, which was really high. For some reason, the bots were really out in full force in that network.
We saw similar kinds of numbers with Brexit, but given the way the network was connected, the bot activity levels, and how the people’s opinions were distributed, the bots didn’t really have any impact on opinions in the network. They were getting drowned out by everybody else talking.
But with gilets jaunes, they had a huge impact. The effect of the exposure to the bots was by five or six times stronger than Brexit. The bots pushing the anti-Macron government message dominated that discussion.
Gilet jaunes was an example where we took the tools we developed and applied them in a foreign language. We don’t read French that well, but we can still measure the opinions using neural networks. In fact, our tools can be applied in any language, which make them very useful in a lot of situations.
Once you have this understanding of what’s happening, how do governments or the platforms or individuals respond?
If you find a bot account, they should be shut down. This is already happening on the social media platforms, but I think they’re missing a lot of bots. Whatever they’re doing isn’t catching all the artificial accounts. So they should be shut down and that should be done more effectively.
The second thing is, if there are bots in the network, then we need a way to understand if they are really affecting people. Just saying there’s a bunch of bots might actually help do the job of the bot creators. Let’s say that I create a couple of thousand Twitter accounts that are bots and put them into a foreign country like, say, Spain. And then the media says, “We found a bunch of U.S. bots in Spain.” Just reporting that fact makes the people distrust everything they’re seeing. It creates a cynicism in the population, which is what the bot creators want. The bot creators want you to not trust what you’re seeing, not trust the news, not trust what you’re reading.
Our method gives a way to assess, for a given discussion topic, how much impact the bots are having. If it’s small, maybe the media shouldn’t report it. It’s just going to create panic unnecessarily. If there’s a significant impact, report it and shut it down. But if there isn’t, you’re just doing the bot creators’ work for them.
You can also think about using bots offensively. If the bots are attacking the U.S., one way to counter is to shut the bots down; another way is to actually put your own bots into the network to counter what those bots are doing. Then my tool gives you a way to assess if your countermeasure is successful or not. Are you hitting the right people? Are you offsetting the damage of those bots?
In another paper we wrote, we looked at how to target people to counter message against a bot campaign. We found a way to identify high-priority people for a bot to counter message what’s being said by the bots out there.
It sounds like if you were on the wrong side, you could be a very effective bot maker.
Maybe. This is a problem I’ve been thinking about for a while. In the 2016 election the Russian bots you hear about in the news just went out there and randomly followed people and posted stuff. I don’t think it was a very sophisticated effort. Had I been doing it, I could have allocated the resources to target much more effectively, to create a much more substantial impact on the sentiment of the voters, and affect the election. Maybe my bots would go to Michigan or Pennsylvania. Or target people who are Bernie supporters in these states and make them think Hillary cheated them so they’ll stay home. If you understand the country’s layout and electoral politics, you can really do some damage.
Are you looking at other issues where bots might be having an impact?
We just completed a study of bot impact on the impeachment of President Trump. We created a website so people can see some of the details of our analysis. We found that there was a small fraction of bots, and most of the bots were anti-Trump. After applying our assessment tool, we found that even though the pro-Trump bots were outnumbered nearly five to one, they were able to have a larger impact some days than the anti-Trump bots. This just goes to show that when it comes to bots, numbers aren’t everything. You need to consider the connectivity, activity, and opinions of the network they operate it.
We are currently applying our bot assessment tools to the 2020 election and we will see what we find.