Putting AI on Every Team
Artificial intelligence is suddenly making headlines. Is the technology ready to become a standard business tool? Bryce Hall ’12, who advises companies on digital and analytics transformations at McKinsey, says that combining human expertise and judgment with AI’s data-driven recommendations is a challenging but powerful way to deliver business results.
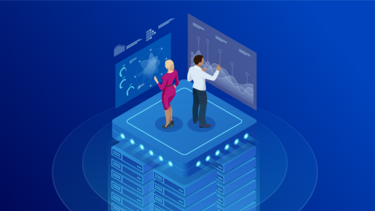
Q: How quickly are companies adopting AI?
In 2017, about 20% of companies responding to McKinsey Global’s AI survey reported adopting AI in at least one of their business areas. Today, that number is two and a half times larger.
However, we have seen a leveling off around that 50% level over the past few years. An important reason for that is that adopting AI isn’t simply investing in a new technology. We see the best results when people can supplement their expertise with the rich insights AI can deliver. One of the core challenges companies face is developing processes to integrate their people and AI tools and insights.
Q: What are the typical uses of AI today?
The top use cases of AI overall are for service operations optimization. Product or service development is consistently near the top. Marketing and sales use cases are too. In all of these, the AI is embedded in products used for things like customer service analytics, customer segmentation, lead generation, new customer acquisition, or marketing.
AI is great with multi-variable optimization challenges. It can take in vast data sets and a vast number of variables and deliver recommendations. For an airline, that might be what passenger routes to fly, improvements to maintenance operations, or how to maximize cargo yield. For a mining company, an AI engine drawing on IoT (Internet of Things) sensors can deliver guidance on precise adjustments to crushers or chemical baths based on the characteristics of the ore being processed. This has enabled mines to increase throughput and yield by more than 10%.
These are incredibly capital-intensive operations, so making them more efficient and more environmentally friendly, also can mean hundreds of millions in savings.
Q: I’m guessing airlines and mines aren’t using off-the-shelf AI.
To date, AI tools have been most naturally suited for an enterprise setting—a large corporation with a large data set and the resources to develop, to train, and fine-tune AI models. And many AI capabilities still require a large team of PhD data scientists, but cloud-based, SaaS [software as a service] AI solutions are expanding quickly and are commercially viable in a broader set of contexts. More and more AI capabilities are designed to involve low code or no code.
Recently, we’ve seen an explosion of public recognition of AI through the generative AI capabilities. The democratization and increased accessibility of AI capabilities has led to a step change in awareness and excitement around the opportunities and also the risks associated with AI.
With the advent of electricity, an extraordinary number of adjacent possibilities were opened up for businesses, homes—all of society. People have suggested we’re now at a point with AI where the adjacent possibilities can expand rapidly.
At Yale SOM, I loved the Innovator class. A concept that has stuck with me is the innovation funnel. To get to a stellar breakthrough idea often requires starting with a broad set of possibilities that are narrowed down to the truly transformative one.
With millions of people exploring the possibilities of generative AI technologies and products for example, that definitely expands the top of the innovation funnel. Many of these users of AI will come up with new ideas and creative applications of AI. To me that suggests significantly increased growth of AI over the next three to five years.
Q: Is this an inflection point?
Time will tell, but I think we can at least describe it as a commercial threshold moment. Previously there was lots of potential, but many AI tools weren’t commercially viable, even if some were put out into the world. Most of us have had an incredibly frustrating experience with an automated call center that used AI in conversational customer support. Now many more applications have passed a threshold where it’s easy to see that they are actually quite useful—and the opportunities just open up from here.
Another concept that has stuck with me from the Innovator class is “the adjacent possible.” With the advent of electricity, an extraordinary number of adjacent possibilities were opened up for businesses, homes—all of society. It wasn’t necessarily electricity itself that was so transformative, but all the applications it enabled. People have suggested we’re now at a point with AI where the adjacent possibilities can expand rapidly.
We’re at this threshold because the AI tools are more sophisticated and more accessible. That has happened because many other trend lines have moved in the right direction—falling cost of data storage, rising data accessibility, development of low or no code applications, tools that enable an AI model designed for a specific purpose to be retrained for adjacent opportunities rather than starting from scratch for each new use. All of that means we’re moving towards assetization and modular components that can be tuned and more easily transferred to a broad range of other applications.
Q: AI has shown it has enormous potential. There’s now extraordinary public interest. Are there are also reasons to be cautious with AI?
Over the past five years, we’ve tracked a set of risks, including cybersecurity, regulatory and compliance, privacy, and bias risks.
It’s a fascinating time to be working in this area because of the growth trajectory and the value that AI can unlock for companies. And it’s a fascinating time because there are so many very real issues that will need to be worked through.
Now, with generative AI, the question of risks come to the fore in a way that is accessible to a broad audience. We’re seeing issues of ownership and copyright, plagiarism, and accuracy—things created by generative AI are sometimes completely inaccurate. And AI can be used in ways that pose real risks. Companies and organizations are now in the early stages of identifying all the risks and then properly mitigating them.
It’s a fascinating time to be working in this area because of the growth trajectory and the value that AI can unlock for companies. And it’s a fascinating time because there are so many very real issues that will need to be worked through.
Q: You started by saying that the best results come from AI and people working together. Would you expand on that?
In most business settings, an AI technology solution alone isn’t sufficient to capture value. AI offers data-driven insights, but people often need to do the real-life decision-making. We have to have people with technical expertise and industry knowledge and combine that with technology capabilities.
Companies that are successfully capturing value from AI spend more than half of their time and investment on the integration of the people and the AI tools. That means things like training employees, tracking KPIs, having a performance infrastructure to unblock issues that arise so that the AI tools deliver accurate useful information and the people put that information to use in a way that gets to the outcome that’s intended.
Companies that get good at this go from idea to proof of concept to implementable solution very rapidly. They also consistently have an executive team aligned on the AI strategy, a clear and quantified view of priorities, and a realistic understanding of the specific tech talent roles and responsibilities required to implement.
Q: Do you ever foresee a day when McKinsey will give clients the option of choosing either a human or an AI consultant?
Just as with other companies, McKinsey has found the partnership between AI and deep human experience and expertise is what characterizes successful outcomes. Sometimes we describe that as hybrid intelligence, and for the foreseeable future we expect that partnership to be needed to get to the best answer.
Q: Does that imply that McKinsey teams are using AI tools?
Absolutely. Consulting has been and is being disrupted by these technologies. We’ve made a broad set of changes and investments to be able to evolve and meet our client needs in this new world.
Today, McKinsey has thousands of colleagues with very deep technical expertise—data scientists, data engineers, software and cloud engineers, UX/UI designers, and consultants—whose primary role is to help clients build and implement data and analytics capabilities. We recently acquired Iguazio to expand our ability to help our clients scale AI, using Machine Learning Operations (MLOps).
One of the cleanest analogies that I’ve heard is if you were remodeling your kitchen, you wouldn’t do that without an electrician, a plumber, and someone to install your cabinets. All the separate specialties are needed to get to the finished product, but you don’t need them all there the whole time.
It’s an exciting time as we’re working with a transformative set of technologies that mean we’re all adapting. That’s true for McKinsey as much as for our client companies.