Can AI Help Design a More Appealing Car?
Designing a new car is expensive and time-consuming—and there’s always a risk that a novel design won’t connect with consumers. In a new study, Yale SOM’s Alex Burnap shows how machine learning can identify promising models and help designers generate new designs to iterate on their ideas more quickly.
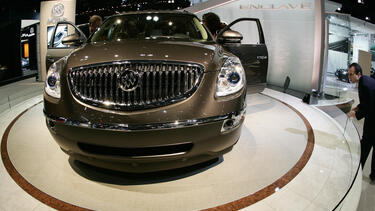
The newly announced Buick Enclave at the 2006 Los Angeles Auto Show.
For automakers, the look of a new vehicle is far more than window dressing surrounding the drive train. Design elements—the body shape’s proportions and color as well as more subtle elements like the interplay between the headlights, grille, and bumper that makes up the “face” of the vehicle—collectively explain as much as 60% of customers’ vehicle purchases. Successful redesigns, such as the Buick Enclave over the Buick Rendezvous, can drive 30% higher prices, leading to billions of dollars of increased revenue. In contrast, aesthetic flops like the Pontiac Aztek have been chalked up to market failures, with billions in losses.

From left, the 2005 Pontiac Aztek, the 2007 Buick Rendezvous, and the 2008 Buick Enclave. The three products had the same engine and drive train but different designs, and dramatically different performance in the market.
Yet designing a new model is both time-consuming and extremely costly, as Alex Burnap, an assistant professor of marketing at Yale SOM, knows firsthand. Working in product research at General Motors earlier in his career, Burnap saw how vehicles evolve from rough sketches on a designer’s notepad to 2D image renderings to life-size clay models complete with functional headlights—and he saw the enormous amount of time and money that companies pour into this design pipeline. ”On average, it takes up to five years and about 3 billion dollars to develop a redesign or new model,” he explains.
It left him wondering whether machine learning could help streamline and augment that process. “Could we do it better, faster, cheaper?”
In a new paper, Burnap, along with John Hauser of MIT and Artem Timoshenko of Northwestern University, explore how AI can help to augment certain time- and cost-intensive parts of the vehicle design process. The machine-learning model they developed has two components: one that could empower designers to more nimbly experiment with new design concepts, and one that could help marketers choose where to focus their efforts.
After all, given the immense cost of producing a life-size vehicle model, product and market researchers cannot simply A/B test different prototypes to see which resonate best with would-be customers. Instead, they rely on “theme clinics,” extensive focus-groups wherein hundreds of highly-targeted consumers are brought in to evaluate vehicle designs on paper. Input from these clinics can tell the marketers whether consumers view a particular concept as “aggressive,” “modern,” or “luxurious,” for instance, which in turn helps them choose a design that will appeal to their desired market segment.
Yet given all of the preliminary work required to develop and flesh out designs, and with each of the hundreds of theme clinics run each year, across different products and market segments, costing around $100,000, companies inevitably spend huge amounts of cash and manpower developing designs that fall flat with the focus groups and are then scrapped.
While working with design teams, Burnap saw an opportunity for AI to improve this process. The idea has two parts, he explains: first, train an algorithm to predict how a human focus group would rate a given design, allowing designers to eliminate the less viable concepts before the theme clinic, “so that you don’t have to kill them further down the process,” and second, use the algorithm to also generate new approaches, to help designers creatively explore the space of possible designs.
To create the algorithm, the researchers set up a deep neural network to determine how features of an image translate into ratings. GM had provided them with 7,000 images of 203 vehicles, plus consumer ratings from focus groups where those vehicles had been evaluated, which they could use to train the neural net.
But there were some major obstacles. First, the researchers had to make due with “small” data: 7,000 images was not nearly enough to train a machine to reliably and accurately predict how images of designs translate into human ratings. To address this issue, Burnap and colleagues beefed up their training data using a separate set of 180,000 unrated images.
How could unrated images help to predict consumer ratings? As Burnap explains, “the idea is to let the ‘big’ unlabeled data do the heavy lifting of ‘learning’ how a product looks, while letting the ‘small’ labeled data focus on how consumers would respond to that product.” For example, before their neural net could learn which body shapes tend to be viewed as appealing or innovative, “first it had to learn that body shapes like SUVs or sedans even exist.”
The next obstacle: Even a single average-sized image is comprised of millions of pieces of information—and all of those variables need to go into a predictive or generative algorithm. For example, a 1,000 pixel x 1,000 pixel grayscale image is a matrix of 1,000,000 variables, far too many to put into conventional predictive models. With so much data, algorithms struggle to discern which features matter and which don’t. To solve this, the team developed a novel encoding algorithm that boiled down each image to a more manageable size without losing key features.
By bolstering and encoding the training data in this way, the researchers’ neural net was able to draw meaningful connections between vehicle attributes and focus group ratings. In fact, their predictive model correctly predicted how consumers would rate an image 30-40% better than other state-of-the-art models.
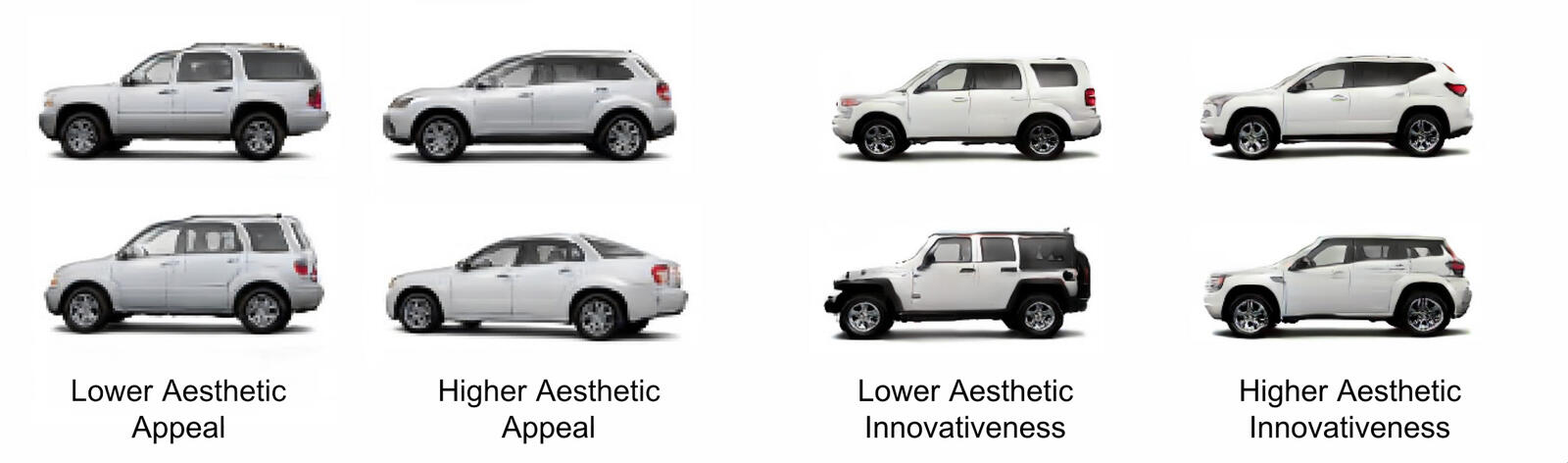
Ratings of vehicle designs from the predictive model. Image: Alex Burnap.
Beyond predicting consumer ratings, the algorithm is geared towards generating “new to the world” conceptual designs that could bolster the vehicle design process. Designers often run the risk of “design fixation,” in which only narrow subset of possible design concepts is explored and focused on. The idea, Burnap says, is to give designers a tool that improves how they develop products, by allowing exploration and early feedback on potential market acceptance.
While plenty of existing AI tools can take a group of pictures and spit out new images that are visually similar, Burnap and colleagues sought to go further, building a generation engine that would incorporate live input from human designers. “Most machine-learning advances in this area focus on generating as realistic-looking images as possible. This turned out not to be the actual need of designers,” says Burnap. “The goal was not to make pretty-looking car pictures.”
Rather, the goal was to give designers greater control over the output, says Burnap. “We wanted to provide different tuning knobs to adjust the elements that are actually important to designers.” These two elements, control and realism, “come at a tradeoff from a machine-learning standpoint,” Burnap notes. “While realism is important, more important is ‘real enough’ but very controllable.”
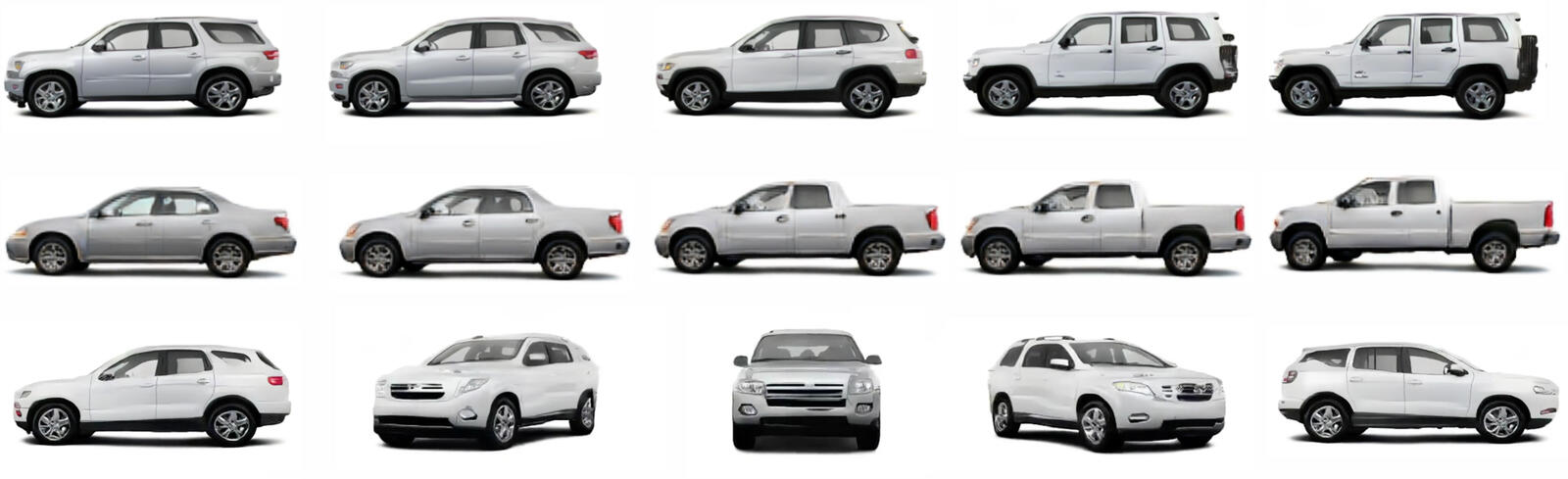
Design concepts created with a controllable machine-learning algorithm. Image: Alex Burnap.
The researchers built on components from several existing models and developed new machine-learning techniques to build an algorithm that allows designers to experiment with various design attributes. “For instance, you could say ‘I want a vehicle that’s half car, 30% SUV, and the last 20% to be truck-like,’” he explains. What’s more, designers can use this tool to “morph” between different attributes—one might turn the “sporty” knob up or down, for instance, and watch how the resulting image shifts before their eyes.
The hope is that the resulting images will be a source of inspiration for designers. “They might say, ‘I never thought of that, but it gives me another idea to explore” Burnap explains. When shown AI-generated images, a group of veteran designers from GM observed that the images, which had been calibrated for high “innovativeness,” were indeed reflecting current design trends—and they were soon discussing how they could build further on what the model had generated.
The algorithm proved remarkably adept at not just incorporating design trends but anticipating them. Trained only on vehicle images from 2010 to 2014, the algorithm was capable of generating designs that matched those of new vehicles released in model year 2020—more than five years into the future, a typical target for design teams. Burnap says, “While this is of course a limited test, given that we can’t A/B test billion-dollar product launches, it does give evidence the approach can creatively generalize into the future.”
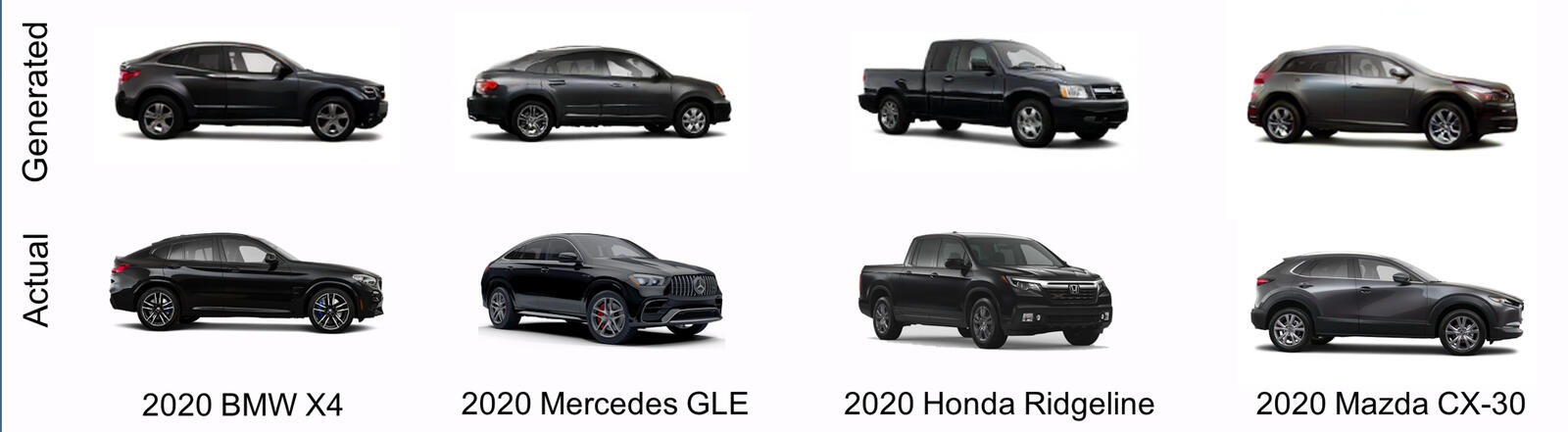
Four designs for 2020 models generated using data from 2010 to 2014, and the corresponding models that were actually released in 2020. Image: Alex Burnap.
That said, both prediction of consumer response to a new design and generation of new designs are aimed at augmenting—not automating—how real design teams work, Burnap stresses. The goal of this work is not to replace human designers, but rather to give them more room for creativity, faster iteration cycles, and higher throughput of designs in both concept design generation and concept design testing, much in the way that the advent of computer-aided design in the 1980s empowered design teams to focus on exploring design concepts in two-dimensional and three-dimensional space, rather than requiring the conversion of sketches to full-size clay models. That’s an important step at a time when most machine learning in the automotive industry is still happening under the hood.
“Design is a fundamentally creative process that both listens to the market but also endogenously guides the market itself,” he says. “We are likely currently at the stage of AI where it is likely most effective to augment and improve how existing workflows are done, not replace them entirely.”