Can AI Help Identify Persuasive Salespeople?
What makes a good salesperson? For a new study, Yale SOM’s K. Sudhir and his co-authors used machine learning to break down effective persuasion into elements like body language, vocal inflection, and spoken words. Their model was able to identify effective salespeople, though it performed best with the involvement of a human judge. The work could help companies consider a wider range of candidates when they are hiring for a sales role.
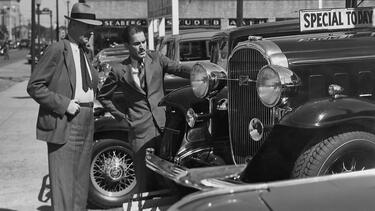
Persuasiveness is a key skill to look for when you’re hiring a salesperson—but it’s also hard to define. While most people can identify a persuasive sales pitch when they see one, even scholars of persuasion struggle to agree on the precise elements that make such a pitch effective.
K. Sudhir, the James L. Frank ’32 Professor of Private Enterprise and Management and professor of Marketing at Yale SOM, suspects that, at least in part, this lack of consensus within the research on persuasion is due to the methodological approach used in this research area, which tends toward self-reporting and surveys to suss out when persuasion works. “It could be that the mixed results we see in the literature are a result of not having truly objective data,” he says.
So Sudhir was excited when Khai Chiong of the University of Texas at Dallas approached him and Ishita Chakraborty, then a PhD student at Yale SOM and now at the University of Wisconsin-Madison, with the possibility of analyzing video recordings of “sales plays” in the format of the National Collegiate Sales Competition (NCSC). In these roleplays, aspiring salespeople participate in mock sales pitches, with the chance to earn an entry-level sales job at an actual firm. Sudhir’s previous research had studied how to incentivize and design sales roles. With the video recordings, he saw an opportunity to conduct an unprecedentedly granular study of the language, voice, and body movement components of effective persuasion, which is critical for sales success.
In a new paper, Sudhir, Chiong, Chakraborty, and Howard Dover, clinical professor and director of the Center for Sales at University of Texas at Dallas tested whether they could leverage machine-learning models trained with body, language, and voice data to predict how human judges would evaluate the persuasive abilities of the subjects in the NCSC roleplay videos. They found that the AI model achieved a reasonable predictive accuracy of 40% improvement over random selection. But by incorporating the input of just one human—what the researchers call using an AI-human hybrid model—the predictions improved to 67% over the random baseline.
Hey, I’m AInsights
Ask me questions about the article and its underlying research. Let’s chat!
The first step for Sudhir and his co-authors was to figure out how to atomize the subjects’ language content, voice inflections, and body movement using AI.
“We thought that if we solved all of these issues, that could be the foundation for a lot of other people to build on in this persuasion literature,” Sudhir says.
The European Union and several U.S. jurisdictions deem the use of AI applications in hiring to be high-risk, as opposed to low-risk uses like AI for marketing. The EU’s AI Act requires that the frameworks of such AI applications be explainable, and Sudhir and his fellow researchers built their model to comply.
“We didn’t want to run a black-box model where we’d say, ‘Here is all the input that goes in, and out comes something magical.’” Sudhir says. “Given the risks involved in AI, it is critically important to be able to explain the decisions to anybody, and it should be reasonably auditable. Otherwise, how do you ensure that no one is being discriminated against?”
That meant the researchers needed to define each of the components of body movement, language, and voice modulation that they hypothesized would factor into persuasion—and then find a way to measure each directly. Importantly, they needed to perform this observation in a context that closely approximated a real-world sales pitch.
The videos of roleplayed sales pitches worked particularly well for this purpose. The researchers trained their AI model using 195 of these videos, which involved university students being interviewed by sales professionals from actual firms. In the videos, students (interviewees) worked to persuade the corporate representatives (interviewers) to purchase a software product. Because the interviews could lead to jobs, both sides were motivated to closely mimic an actual sales pitch.
The researchers broke the features they needed to track into three categories often delineated in the sales persuasion literature: content (what is said); style (how it is said); and conversational interactivity and “matching”—whether an interviewee subconsciously absorbed and made use of the style and language of the interviewer. They gathered information on subjects’ language use, body movement, and voice to flesh out each category.
AI is more consistent. But the human is detecting some things that the AI is not detecting, at least in this current stage of AI. So the AI-human hybrid is superior to the AI or the human alone.
For example, the researchers broke down the “content” category by capturing how many spoken words each interviewee devoted to broad topical areas like greetings, product, and pricing. They also tracked overall word counts to serve as a proxy for the information density of the pitch.
The “style” category was more complex, as the researchers sought to investigate verbal, body-language, and vocal styles. To assess verbal styles, the researchers used an AI-assisted process called “sentence embedding” which allowed them to measure how closely the interviewees’ words hewed to example sentences displaying the traits of collaborativeness, confidence, and politeness. “A particularly interesting variable that we constructed,” Sudhir notes, “was how effective interviewees were in adapting to the language styles of the interviewer—this adaptability turns out to be important in persuasion.”
To study body movements, the researchers used OpenPose, an open-source tool that can track specified points on human faces, bodies, and hands in two-dimensional images and convert them to data. The tool allowed them to extract pixel coordinates of 24 body parts from every frame of their videos. Using this low-level coordinate data, the researchers built higher-order body language signals, like the rate of hand gestures and head movement, which allowed them to assess the confidence or nervousness of the speaker.
All of this rich, multimodal information fed into the AI model’s predictions of who displayed the strongest persuasive powers. Since each of the videos had been scored by a panel of nine judges with corporate backgrounds similar to those of the interviewers, the researchers could compare their model’s picks with those of trustworthy human scorers.
Perhaps the most interesting finding from the research, Sudhir says, was the indication that the AI-human hybrid model did the best overall job of choosing job candidates based on their persuasive abilities.
“The AI is more consistent. But the human is detecting some things that the AI is not detecting, at least in this current stage of AI,” Sudhir says. “In that sense, the AI-human hybrid is superior to the AI or the human alone.”
It also turned out that some of the multimodal features of the conversations were more important than others. The content of what was spoken reigned supreme in determining persuasiveness—though conversational interactivity, interviewees’ real-time adaptation to interviewers’ styles, and body language also played key roles.
Sudhir acknowledges that there are legitimate concerns about the use of AI in hiring. Still, he argues that this type of AI tool could be a breakthrough for hiring entry-level candidates in sales jobs, where persuasion is a critical skill. Résumés don’t sufficiently distinguish between candidates with sparse work experience, and traveling to colleges and universities to interview soon-to-be graduates is expensive and inherently exclusive.
“We don't think about the hidden costs of not using AI,” Sudhir says. “If a company doesn’t have AI, they likely only go to the top schools to interview.” By leveraging an AI tool, he explains, the same recruiters could afford to analyze videos of interviews and therefore expand their search to include a broader range of students. “We think these benefits of AI can outweigh the drawbacks.”
He also hopes that his team’s approach and findings can serve as a foundation for new workplace tools, even beyond hiring—perhaps assisting individuals with beefing up their powers of persuasion, for example.
“We could train the AI’s ability to convert videos into something meaningful and then help people get feedback,” Sudhir explains. “There are all kinds of applications here that can build from this as a starting point.”