In Defense of (Mathematical) Models
How many people are likely to die from COVID-19? How many will need to be hospitalized? Epidemiological models have helped answer questions like these and played an influential role in governments’ responses to the COVID-19 pandemic. At the same time, they’ve been attacked as alarmist by critics of restrictions. Yale SOM’s Edieal Pinker takes a look back at one of the most influential models and argues that such rigorous efforts at understanding the likely course of the disease, while imperfect, are critical to good decision making.
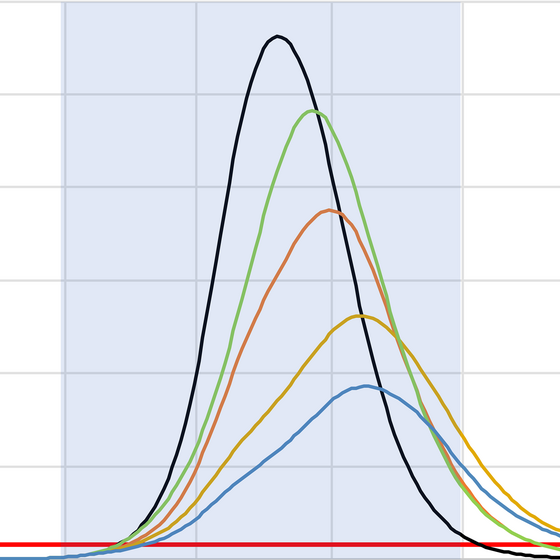
A chart of ICU occupancy under various scenarios from Imperial College London
During the COVID-19 pandemic, Americans have probably heard more about models—mathematical ones, that is—than at any time in the history of the country. Such models use epidemiological principles and data about the disease to make projections about the likely course of the disease. They have been used on many different geographic and time scales to make projections about the number of COVID-19 cases and deaths, the demand for ICU beds and ventilators, and the impact of possible interventions, among other factors. The outputs of these models have informed decision makers and helped public health officials and healthcare systems make operational plans. The models have also been used as ammunition in political conflicts and as a result come under much criticism.
The attacks on models are unfortunate because, used well, mathematical models, and in particular epidemiological models, can be very valuable tools. Dismissing them as mere speculative exercises contributes to an alarming trend of dismissing science and the expertise of scientists altogether. This only makes us less informed.
In this essay I hope to counter some of the loss of confidence in mathematical models by focusing on the model of the COVID-19 pandemic that was developed in March of 2020 by a team at Imperial College, London, led by Dr. Neil Ferguson. This model received an enormous amount of attention, influenced policy responses to COVID, and has become a target of much criticism since. For those readers who are unfamiliar with it, the Imperial College model is the one that projected that 2.2 million Americans (and 510,000 residents of the UK) could die from COVID-19 over the next two years without public health interventions. The projections were credited with spurring government leaders to take strong actions to stop the spread of the virus—including restrictions on activity that shut down large parts of the economy for several months. Critics of these actions have characterized them as alarmist overreactions to an unrealistic doomsday scenario that were perhaps politically motivated and underweighted their economic and social costs.
There are a number of questions we can ask about such a model. How accurate were its projections? What was its purpose? What did the model show? What influence did it have? Before answering these questions, it is important to take a step back and set realistic expectations for this kind of modelling.
“A model of such social processes can never achieve the accuracy of a physics model of the motion of a projectile, because the system being studied is adjusting to its situation in real time.”
The COVID-19 pandemic is a one-time event. While it is similar to previous events like influenza pandemics and likely similar to future pandemics, it is the only one with its specific characteristics occurring in a particular human environment. When we try to develop a model that serves as an accurate representation of its dynamics, we are attempting to use all the available scientific knowledge about what has occurred up until now to inform us about what could happen with this virus. An epidemiological model includes biological processes of a virus interacting with human immune systems as well as social processes of people interacting with each other and making decisions to protect themselves from the virus. A model of such social processes can never achieve the accuracy of a physics model of the motion of a projectile, because the system being studied is adjusting to its situation in real time. In other words, human beings adjust their behavior—in a way air molecules and bullets do not—as they learn more about a disease or other threatening situation.
What was the purpose of the model? One purpose was to determine how many lives are at risk from this virus. In other words, how worried should we be? To demonstrate how this works, we can construct our own simple model. According to the CDC, the 2017-18 influenza season was particularly bad, with perhaps 45 million Americans getting sick and 61,000 dying. One could estimate that COVID-19 is much worse than a seasonal flu and thus twice as many will get it and die as a result. That is also a “model,” but one that is using arbitrary parameters: take 2017-18 as a benchmark and multiply by two. If one believes the parameters are reasonable, such a simplistic model might be useful, giving government officials an appreciation for the seriousness of the pandemic they are confronting. But such a model leaves out a lot of information. Over what time periods would we see the cases and deaths? What would the load on hospitals be? What would be the impact of limiting contact between people for some period of time? These are important questions to answer if you want to take actions in a timely way. The Imperial College model attempted to address exactly these questions.
In the media, the outputs of the model were portrayed along the lines of this example from the New York Times on March 17: “The report, which warned that an uncontrolled spread of the disease could cause as many as 510,000 deaths in Britain, triggered a sudden shift in the government’s comparatively relaxed response to the virus. American officials said the report, which projected up to 2.2 million deaths in the United States from such a spread, also influenced the White House to strengthen its measures to isolate members of the public.“
This is not exactly what the report said. Rather, it said that short-term mitigation efforts would reduce potential deaths in the U.S. by 50% to 1.1 million over a two-year period. This point was seized upon by more conservative voices to say that the threat had been overhyped. On March 27, the Wall Street Journal editorial page said of the Neil Ferguson team report: “Much of the public attention focused on his worst-case projection that there might be as many as 2.2 million American and 510,000 British deaths. Fewer paid attention to the caveat that this was ‘unlikely,’ and based on the assumption that nothing was done to control it.” This begs the question, would 1 million deaths be acceptable to the WSJ editorial board?
What has happened since March of 2020? We are about seven months later and in the U.S. there have been more than 250,000 excess deaths—a death rate that is not that far off from 1.1 million over two years and much, much worse than the seasonal flu. Thus the much-maligned Imperial College projections do not seem so extreme. They are certainly not comparable to asteroids that never impacted the earth—the parallel drawn in a WSJ op-ed on April 1 by Benny Peiser and Andrew Montford.
The model also projected that hospitals would be quickly overloaded if the pandemic was unmitigated. Not only did this spur public health efforts but it also spurred preparations by hospitals that enabled them to expand their capacity to handle the flow of patients. Our own Yale-New Haven Hospital, a facility that normally has a total of 1,500 beds and runs at 90% bed utilization, was able to handle at its peak 460 COVID patients. This was possible because of advance warnings given by models.
“If the Imperial College model indeed spurred the government, healthcare systems, and individuals to take action, then it made a great, even heroic, contribution to society.”
So if the Imperial College model indeed spurred the government, healthcare systems, and individuals to take action, then it made a great, even heroic, contribution to society. In fact one could argue that the observation of lower-than-predicted numbers of deaths demonstrates the success of the modeling effort and the benefits of the societal response.Another output of the model was to show what to expect if mitigation efforts were carried out for three months at a time. Below is a drawing from the report showing weekly ICU cases in the UK if the restrictions were lifted after three months—that is, in July. In many jurisdictions in the U.S., restrictions were lifted in the early summer, following a policy of a few months of strict measures followed by loosening that is similar to that depicted in the Imperial College report. We can compare the Imperial College projection to a graph of new COVID deaths—which should follow a similar pattern as ICU cases—reported in the U.S. (from the New York Times website on October 13, 2020). In fact, qualitatively we see that deaths rose through the late summer in the U.S., as could have been inferred from the graph generated by the model.
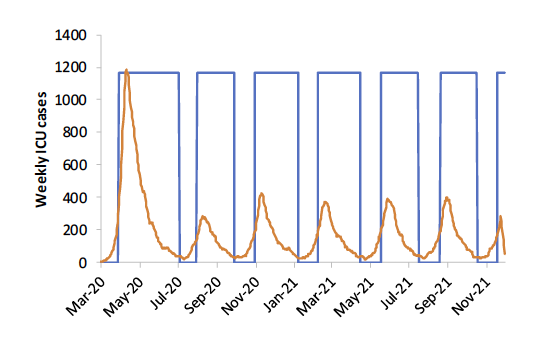
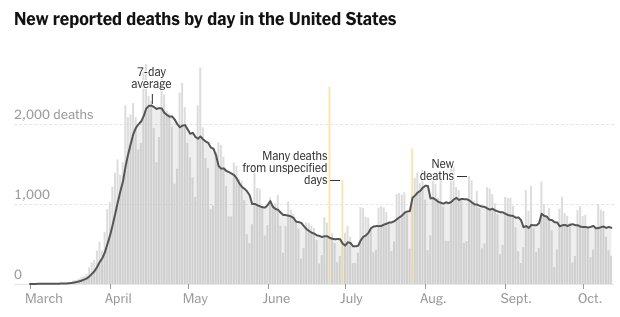
This is not to say that models are perfect or that they are always used appropriately. One reasonable concern about the Imperial College model is that it is too much of a black box. While the research team reported many of their assumptions, those assumptions were inputted into a complex computer microsimulation that they have used in the past for other epidemics but that is not open source. As consumers of the results, we had to rely on our confidence in the expertise and reputation of this research team because it was not feasible to directly examine their code. On the other hand, other epidemiologists could take their assumptions and create relatively quick versions of the standard SEIR (Susceptible-Exposed-Infectious-Recovered) model that provide sanity checks on the work. They did and found broadly similar results (see, for example, this paper by Yale SOM’s Edward Kaplan).
Another problem with these kinds of models is that regardless of their sophistication they rely on inputs that are uncertain because the virus is a new phenomenon. For instance, basic variables, such as the disease’s infected mortality rate, were only roughly estimated early in the pandemic’s spread and are still being studied. But what is the alternative? Uncertainty about the contagiousness, lethality, protection methods, and treatment options are inescapable and our understanding is evolving over time. Should we just guess instead? A model gives us a structured way to organize the limited information we have about the virus and identify what forces are most important to the outcomes. The structure should be based upon accumulated knowledge about similar phenomena thus far—and logic.
As we’ve seen, the Imperial College model provided a reasonable sense of the magnitude of the threat from COVID-19 and useful information about how public health efforts could mitigate its harm. Model-driven policymaking in these uncertain situations makes us less likely to make big errors and thus buys us time to collect data and improve our understanding of the unknowns. For COVID-19, medical professionals and the pharmaceutical industry have used this time to develop better treatment approaches that reduce mortality. We get closer to a vaccine every day. We also collect data that indicate public-health interventions that are more targeted and efficient. For example, a recent study from a Yale colleague shows the potential benefit of restricting movement of staff across nursing homes—a problem whose scope was not known to us in March 2020. This time also allowed other colleagues at Yale to develop a model for determining good testing protocols for students in residential colleges, facilitating the reopening of institutions of higher education.
Early in the pandemic, some commentators dismissed policy models, arguing that the economic costs of restraining social interaction were too great compared to the health risks—which they insisted on downplaying with little data. Policy modeling capability is an asset to our society because it gives a framework for informed debate. It draws on what we’ve learned from studying the underlying social and biological dynamics of disease. Rejection of models allows politics to have free rein, resulting in policy determined purely by power, influence, and eloquence.