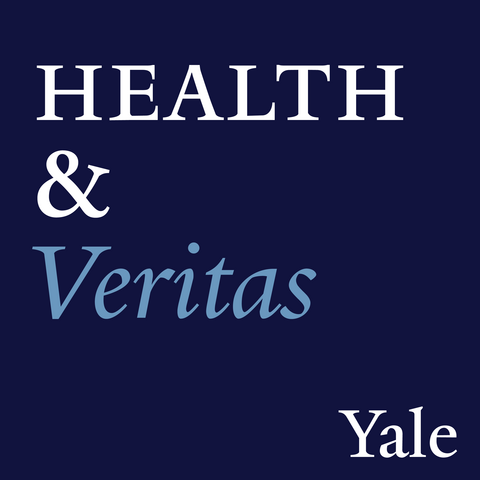
Edward Kaplan: What Does Wastewater Reveal about COVID-19?
Subscribe to Health & Veritas on Apple Podcasts, Spotify, YouTube, or your favorite podcast player.
Howie and Harlan are joined by Yale operations expert Edward Kaplan, who uses mathematical models to understand a variety of processes. They discuss Kaplan’s ongoing research tracking COVID-19 infections through sewage, which bypasses the biases inherent in data from testing and even hospitalization.
Transcript
Harlan Krumholz: Welcome to Health & Veritas. I’m Harlan Krumholz.
Howard Forman: And I’m Howie Forman. We are physicians and professors at Yale University. We’re trying to get closer to the truth about health and healthcare. This week, we will be speaking with Professor Ed Kaplan, but first, I want to get to a topic that broke in the news involving the governor of Utah, Governor Spencer Cox. I’m not very familiar with him. He’s a Republican governor. He’s done a seemingly good job managing COVID, but what surprised me today is that he vetoed a bill that would’ve banned transgender individuals from participating in high school sports. He did so for a number of good reasons that he explains in a multi-page letter that we will hopefully link to this podcast, but he also made it on the basis of a public health decision. I’m just going to summarize that ending point by reading from his letter.
He said, “Finally, there is one more important reason for this veto. I must admit, I am not an expert on transgenderism. I struggle to understand so much of it and the science is conflicting. When in doubt however, I always try to err on the side of kindness, mercy and compassion. I also try to get proximate and I am learning so much from our transgender community. They are great kids who face enormous struggles. Here are the numbers that have most impacted my decision: 75,000, 4, 1, 86 and 56.
“Seventy-five thousand high school kids participating in high school sports in Utah. Four transgender kids playing high school sports in Utah. One transgender student playing girls sports. Eighty-six percent of trans youth reporting suicidality. Fifty-six percent of trans youth having attempted suicide.
“Four kids and only one of them playing girls sports. That’s what all of this is about. Four kids who aren’t dominating or winning trophies or taking scholarships. Four kids who are just trying to find some friends and feel like they are part of something.”
I’ll stop there with reading from this article, but I just want to point out, it is a public health argument. It is an argument about making decisions in the public’s best interest, not on an ideological basis, not on a political basis, but based on actually considering the human beings behind these decisions. I would ask everybody to read the full letter, but I am not a Republican. I don’t live in Utah. I could not be more proud of this man’s decision today, and I think it’s the right one.
Harlan Krumholz: Yeah, it would be nice if it set an example to others. Look, you and I both know people who have struggled with this and know how difficult it is, both for the discrimination that they face externally and for the doubts and uncertainty that they have internally as they try to piece through exactly who they are and how they want to proceed among various different paths. To politicize this, to interfere in people’s lives, to make this more difficult—it seems unkind. I really like the idea that this politician is saying he tries to be kind and think about the implications for those individuals so that it becomes less of a political talking point and more about what’s the best path for us as a nation to be able to help support people who are trying to figure out things and whether that has to do with this issue or so many other issues in society. I think we need to step forward with kindness first as we start to think about what should our policies be. I couldn’t agree with you more.
Howard Forman: Now I’d like to go to our guest. I’m excited to introduce Professor Ed Kaplan. Ed Kaplan is the William N. and Marie A. Beach Professor of Operations Research at the Yale School of Management. He’s also a professor of public health. He is also a professor of engineering at Yale. He’s one of the leading experts in the nation and, quite frankly, in the world in operations research. He has made invaluable contributions to public health by applying mathematical modeling concepts to study public health policy. He is one of the very few people in the world who is dually elected: both elected to the National Academy of Engineering and the National Academy of Medicine, or what was the Institute of Medicine. He’s also a recipient of the Lanchester Prize and the Edelman Award, among many, many other awards.
His recent research has modeled the progression of COVID-19 outbreaks through analyzing wastewater systems. But since we’re in the middle of NCAA season right now, even though it’s the Health & Veritas podcast, I want to start off by asking why you did research over 20 years ago and you published it on modeling the NCAA tournament and what you learned from that.
Harlan Krumholz: Why don’t we ask him first who’s going to win? We’re at the Sweet Sixteen, might as well get the inside information on who’s going to win.
Edward Kaplan: The fact of the matter is I’ve stopped following the men’s tournament for the past several years. I’ve only been looking at the women’s game. Of course, I have a vested interest there because I’ve been a UConn fan forever, having been at Yale for more than 35 years now. But what I can tell you is very simple. When I did this, I actually did this with Stan Garstka, who’s the former deputy dean of the Management School. We were always involved in these little office pools that I’m sure you people have played in as well, filling out the brackets, trying to guess as many games correctly as possible or more accurately, try to score as many points as you can in the pool, often doing it for small sums of money, maybe for larger sums of money. Anyway, Stan and I were casual basketball fans, but we always lost these things.
All of a sudden, one day we were talking about it and said, “Wait a minute. This is actually a very, very structured and well-defined mathematical problem. We should be able to do better than just wing it.” So I started thinking about it and actually was able to, for the first time, write down a very careful mathematical description of how these office pools work for a fairly general set of rules. So it’s not just, pick as many games [as you can]. It’s not just, you get more points the more rounds you get right, but you also can have upset points and bonus points, and the upset points accrue if you’ve got the actual team as opposed to you predicted something and something else happened, but it was still an upset, well, all this complicated stuff. So anyway, it turned out that this was, first of all, an amazing amount of fun, and then we actually did quite well in one of the original internet pools that CBS Sports used to run.
Now, it was a big pool then. It’s a small pool now, but there were like 95,000 contestants, and we just pushed the button and didn’t do anything else. We’re coming into the final weekend, and we were in third place. We only had to have one game come in and if that one game came in, no one would be able to overtake us. That game was between North Carolina and Tulsa, and Tulsa being the underdog was in fact the upset pick that our model had chosen. As it turned out, Tulsa lost that game. So when people say, “What have you learned from March Madness?” my answer was, “Don’t bet on Tulsa.”
Harlan Krumholz: How close was the game?
Edward Kaplan: I’m trying to remember. I think it was within 10 points. Tulsa was winning for a while. I had to stop watching and take my daughter to a swimming pool at the JCC because I couldn’t watch. I was too nervous. But I’ll tell you, there is actually one serious note that goes with this stuff, which is, I teach mathematical modeling, and most of the applications that are taught in a business school, they involve operations in industry, manufacturing, services, maybe in finance, and not all students are interested in these topics. So all of a sudden, by having an example that was completely sports-based, the general interest suddenly picked up and people could see not only that there was a way to model these things, but that actually, you could be reasonably accurate relative to just flipping coins and even relative to just picking the seeds.
So suddenly, people might think, “Well, gosh, if these models are working well on something that I think I know about, basketball, maybe we should start paying more attention when you take the same family of methods, but you are applying them instead to problems in public health, their problems in homeland security, problems in urban planning.” So that was the more serious piece that came out of that.
Howard Forman: You’ve done a lot of work that has used modeling to inform public health decision-making, to inform policy making, and so on. One of the things that has struck me over the last several months is that we’re getting less and less data in many areas than we used to because a lot of states, municipalities are like, “COVID is over. We don’t really need to track this as carefully as we used to.” I know that you’ve used very granular data, including wastewater data, which I’ll allow you to talk a little about as well, to inform that. Can you give us a sense of why it’s so important to have granular data about cases and hospitalizations or wastewater in order to help make decisions?
Edward Kaplan: Well, let’s take a step back because you just mentioned a few indicators. You mentioned cases, you mentioned hospitalizations, and you mentioned wastewater. People could also mention deaths, for example, mortality, people care about. I would argue that they all share certain things in common, and that thing they share in common is they’re all consequential measures of something that happened as a result of infection. They are all time-lagged from the moment of infection. But one thing that’s very different is that when you take a look at actual reported cases—the stuff that actually probably gets the most play in the newspaper—that depends as much upon testing policy, testing behavior, reporting policy as it does on the epidemic itself.
And it amazed me—and I was quite distraught about this early on in the outbreak, and Howie, we wrote a little bit about this together; I know Harlan, you were also very concerned because you launched your own seroprevalence survey to try and deal with this—the fact that the testing was, first of all, restricted, and then secondly, that it was not the case that tests were being applied widely to discover infection; rather, it was people who were getting sick were driving the testing. Testing was really being used to verify that someone coming in with serious symptoms actually was infected with coronavirus as opposed to something else. So the beauty of the wastewater, if I can just switch to that for a second, is that you don’t have the same kind of a sampling bias at all. With the wastewater, it’s a pretty consistent sample from the community.
Now it’s a noisy sample in that sense, and that you could have a steady level of COVID in the community and the actual numbers in the wastewater would fluctuate up and down, but they would fluctuate up and down randomly because of the sampling as opposed to being systematically biased away from whatever the true underlying outbreak trends were because of the sampling thing. We actually have a recent analysis that was just published that demonstrates this very point. If you consider the first two waves of COVID in our neck of the woods, if you were to look just at cases, the second wave looks much, much worse than the first. It obviously lasted longer, but also the number of cases that we were reporting was much, much higher. But if you just look at simply how many tests were being done day to day, it increased and increased and increased and increased over time.
When you look at the wastewater, what you discover is that the intensity of those two outbreaks was the same. The second outbreak was longer, but in terms of the intensity, how high the COVID levels in the sludge were, it was about the same. So that first wave was much more serious relative to the second wave than just looking at cases would suggest. Looking at hospitalizations, however, it gives you a better picture. There were a lot of hospitalizations in that first wave. Hospitalizations are better than cases in the sense that if someone really gets sick, the chances are that they’re going to end up in a hospital. It suffers in the sense that there’s a longer lag time, the time from infection until someone actually has symptoms, realizes they’re ill, gets sick enough that they have to go to the hospital. Of course, it’s only a fraction of infections that end up being hospitalized.
But in the sense of a biased look at just ascertaining what’s going on, I would argue that hospitalizations and deaths are both better than cases. They’re both lagged much further behind. So the beauty of the wastewater is that it’s got a shorter lag relative to those things and it also doesn’t suffer from this systematic bias. Today, by the way, where so many people are getting home-tested or doing rapid antigen testing on their own, so plenty of people who are testing positive are not even being reported on the one hand.
Harlan Krumholz: No, no, we’re not getting it at all. And by the way, I would just say, hospitalizations, there’s two things. One is, it’s highly discretionary who gets in the hospital. Of course, at the far end of the spectrum, for severely ill, they’ll get in, but the threshold for admission were changing over time, also changing depending on what was going on with capacity and so forth. Then in addition, of course, antivirals were being introduced.
Edward Kaplan: That’s right.
Harlan Krumholz: You also were getting a changing with regard to the treatment. So I’ve argued for a long time that the hospitalization rate is very noisy and the death rate [too]. So that’s why we developed a new way of doing the excess mortality. It wasn’t that it was so clever, but we took into account taking out the deaths and considering the lack of immigration. We improved upon what the CDC was doing. They ultimately adopted what we were using because they needed to have a better denominator. But I think excess mortality is much better. I don’t trust the labels on this.
There’s an article that came out just today that said there was a spike in cardiovascular and stroke deaths in early 2020, and they want to say that that was the pandemic. Yeah, it was the pandemic. I just don’t know, when you find someone dead in their apartment, what did you label them? There were no tests in the very beginning either, so what was it that people were being called? It’s hard to tell. So we’ve got to go for metrics that don’t have the bias. By the way, what are the current wastewaters saying in the communities across the country? How do you interpret that data?
Edward Kaplan: Well, it looks like it’s starting to go up again. That’s the sad news. Now, of course, there is still variation from place to place. My focus has really been local, as it has been for most of the outbreak. But back to the wastewater for a second. What’s incredible here is that you really do see these outbreaks in the waves just in terms of the concentration of coronavirus RNA in the wastewater. Where we are locally, there have been three very well-defined big waves. The sewage has done what everybody thought Omicron was doing: much more contagious, much quicker on the uptake, and quicker on the retreat, and that’s exactly what you see in the wastewater. You see a very fast up and a fast down relative to the earlier waves. Unfortunately, it’s starting to go up again.
The absolute levels are still very small relative to the large levels that we had. One of the things which is so awkward about COVID is that, on the one hand, it’s been said many times that people are done with COVID, but COVID is not done with us. People are very tired with it, and so they can’t wait until the levels of cases, hospitalizations, case rates, seropositivity fraction, whatever you want to call it, it’s low enough that you can go out and start taking off your mask and go out to restaurants and everything else. But inevitably, what happens is, people overreact when the news is good and then it’s not like these waves come and go on their own, as you know. These waves are created by our own behavior. So right now, we’re at a point in time where again, the mask mandates have ended. People are done with this and yet, we start to see the numbers starting to go up again.
So trying to somehow another synchronize things, if we get out of this constant boom and bust sort of thing, and if we get to a point where actually, all right, this is a disease we’re going to have to live with, we’re not going to eradicate coronavirus, it should be conceivable that it gets to something like an endemic level. We could have seasonal outbreaks like we do with the flu, but right now, it’s like things are bad. We all tuck in. Things look like they get better. Everybody comes out and parties, and that all of a sudden brings on the next wave. It just looks like that whole pattern might be about to repeat again.
Harlan Krumholz: One quick thing I want to ask you about, one thing you’re really known for is this needle exchange thing. In fact, you really transformed the way people think about how to prevent HIV into work in partnership with people and to try to make it work. Can you just say a few words about that program?
Edward Kaplan: Yes, I can. Thanks for asking. As I’m sure your listeners know, needle exchange is an intervention to try and stop the spread of HIV by the sharing of needles, other drug injection equipment among drug injectors. These were very, very controversial programs because on the one hand, they were meant to stop the spread of HIV, which is a good thing. On the other hand, they enabled drug users to continue injecting, which was viewed as a very bad thing by many. The state of play at the time I got involved was that there were a number of studies, all of which relied on self-reported surveys. So you would ask a group of people, “How often are you sharing needles?” Then you’d put them through this needle exchange program for a couple of months where you would say, “Don’t share needles. Don’t share needles.” And then a few months later, ask the same people, “How often do you share needles?” And lo and behold, they say, “We’re not sharing needles anymore.”
Well, of course, you were telling them not to, and so a lot of people didn’t find those surveys credible. One of the nice things about the modeling approach is that it could actually suggest a different way of looking at the problem, suggest data to collect that hadn’t been collected before, and suggest different performance measures to understand how epidemics are being transmitted. So with needle exchange, actually, the suggestion was very, very clear. When people are sharing needles, a person infects a needle, a needle infects a person. Name another disease that has that characteristic. A person gets bitten by a mosquito, and another mosquito bites that person. So you can think of malaria outbreaks where basically, you have people and mosquitoes, and now you have needle exchange where HIV, you have humans and needles. So it turns out that those things actually have a lot in common, as do all kinds of other so-called vector infection processes.
But what that immediately meant is that you could study the needles. You could actually say how many needles are being infected over time. The more infectious needles that are out there, the better the chances that a person using those needles gets infected. What the needle exchange program is going to do is reduce the length of time any individual needle is out there. It’s as if you want to do a malaria epidemic and took all these infected mosquitoes and yanked them out and replaced them with newborns that were free of disease. So suddenly, it suggested a whole new family of performance measures—not only the fraction of needles that were infected but actually the exchange rates and the circulation times of the needles, as well as sharing rates and things like that. We were able to demonstrate by actually tracking the needles themselves that in fact, the circulation times went down. They were only out there for a couple of days at a time, and that all of a sudden makes you realize that the needles are sharing fewer people, right?
Harlan Krumholz: Yeah, and it worked. I just want to say, I thought it was courageous also because the idea of going out and saying, “We’re going to give drug abusers needles,” it was a talking point for lots of people about what was wrong with public health. In fact, it was exactly what was right with public health. You defended it. It showed better outcomes. Of course we want those people off of drugs. Of course we want to help them to address their addiction, but we also want to help them avoid HIV. It’s a double disaster if they get HIV and addiction at the same time. So anyway, I thought that was incredible courage that you went into what was really a very difficult area.
Edward Kaplan: The beauty of this whole thing was not only did HIV go down, but now all of a sudden, you had a group of people who trusted the program. Consequently, many of those people were actually willing to go into drug treatment as a result because they felt that the people involved at the program were actively trying to help them as opposed to trying to coerce them. Consequently, the number of people who actually wanted the drug treatment went way up, and so we won on both counts. The levels of drug use went down, and HIV infection went down.
Harlan Krumholz: You know, Ed, I’ve been admiring your work for such a long time. When I was in training, I spent time, as you know, with Milt Weinstein and one of the also leaders of mathematical modeling, particularly in that case applied to healthcare. I’ve long thought that we should be doing much more modeling in the decision-making the key critical decisions that are facing patients. Now, there was a guy, Steve Pauker, who was at Tufts for many years. He was again, another one of my heroes, Steve, a brilliant intellect who brought modeling to the bedside, who had actually a clinical consult service that you could say, “We’re facing a tough decision with this patient, and it would be helpful if we formalize that decision in terms of the choices, the probabilities,” and so forth.
Now, medicine, as you know, as it’s taught in medical school is like, there’s an answer. So you go to a patient and say, “You need to have chemotherapy. You need to have radiation therapy. You need to be on this drug. You need to be on that drug.” We don’t engage in the real decision-making that would require us to say, “There are two choices. We don’t know what’s going to happen with either choice, but here’s what could happen,” and go down that path with them. Now with technology, it would seem to me, we could transform what Steve Pauker used to do. Have you thought much about how we could transform the clinical landscape by incorporating these models to a greater extent?
Edward Kaplan: Well, it’s actually interesting that you bring this up. I would argue that a lot of this is happening already. It hasn’t necessarily been adopted everywhere, but for some time now, people have [been] interested in, I guess it’s gone by the name of “expert systems” in decision-making problems. While it’s not the case that these things are set up so that you can bring any problem at all under the sun that you might face clinically and say, “What’s the right answer for it?” but there are classes of problems where actually, people have done a pretty good job at trying to assemble all the data, trying to assemble everything that’s known from clinical trials, from doing exhaustive published literature searches, looking at observational studies, and then trying to basically do the kind of techniques that you are mentioning, coming up with appropriate probability distributions for if we do this, what are the likely outcomes? I know that in cancer treatment, for example, there’s been some work that way. I know that in HIV, there’s been some work that way.
Harlan Krumholz: But the important thing about these systems, though, and I haven’t seen this, maybe you can enlighten me a little bit, is that they incorporate the patient’s perspective to the extent... We would talk about this in terms of utilities, but—
Edward Kaplan: Right.
Harlan Krumholz: ... how do I weigh this outcome versus that outcome? How do I feel about bleeding versus a stroke? How do I feel about—
Edward Kaplan: Right.
Harlan Krumholz: ... fates worse than death? What do I feel about what it takes to get there? Because many of the treatments may be noxious or involve a upfront risk. In my field, for example, I can get you bypass surgery, but your risk in the next two weeks is going to be higher than what it would’ve been otherwise. Maybe your downstream risk might be lower. Getting all that into the model and then being able to communicate it to the patient in a way that’s clear, to me, still seems like an obstacle.
Edward Kaplan: Well, it is. If you think about it, this is one of these topics where the theory is really well worked out and the application is lagging by years. The reason is, it’s kind of exactly what you said. If you’re a patient, it can be very, very difficult to even necessarily understand the question, let alone come up with a way of thinking through some of these trade-offs. So if I’m asking myself, all right, how do I value having four remaining years of really, really good life versus 10 remaining years, but the quality of life is not going to be so great? Do I really want to just do things so that I maximize the duration of my own survival, or do I basically want to say, “I want to have as great quality of life as possible, then once that’s no longer possible, I don’t care anymore”? Trying to get people to think that way is very, very hard. As you know, there have been all kinds of numerical scales that have been developed, the quality adjustment type scales.
Some of this is done, a survey approach effectively where many, many patients who are exposed to the same classes of health conditions are asked to make trade-offs of this form. What would you prefer? In other cases, people try to get fancier about it and try to set up lotteries. Suppose you could have this for certain or you could have an X percent chance at this great outcome and a one minus X at this lousy outcome, what’s X that would make you indifferent? So the theory is kind of well set up for understanding these things, but actually implementing it is pretty hard, because it puts a serious demand actually on the patients to think about themselves in ways that maybe they’re not used to thinking about. Frankly, the same types of decision-making problems when instead of talking about patients, you actually come up to policy officials in government and in areas not only in health but in national security or in economic development or what have you, they also have a very, very difficult time making these kinds of judgments.
Harlan Krumholz: The one other issue here to me that seems challenging is, I can ask people about these time trade-offs. I can say, “How would you feel about how many years in perfect health versus years in—
Edward Kaplan: Right.
Harlan Krumholz: ... maybe with some disability?” That still may be different than their revealed preference when they’re actually faced in the situation in the choices—
Edward Kaplan: For sure.
Harlan Krumholz: ... they make. So asking them in the—
Edward Kaplan: How do I feel now versus how am I going to feel in four years? Exactly.
Harlan Krumholz: And what happens when you’re in the moment? I’ve heard a lot of patients who will say, “Don’t keep me alive,” but then when they’re in the moment, they actually have a different preference. So you have to enable people to change their mind and have that agility when they’re actually faced with the situation. I just find these interesting complexities, but I’m a big fan of the method.
Edward Kaplan: One misunderstanding some have, I’m sure you don’t have it, is some people think that models make decisions. The models don’t make decisions. People make decisions. Clinicians make decisions. Clinicians and patients together make decisions. The models provide you additional information that you may not have had otherwise. You could argue that in some sense, all the raw data which these models are using were already there, but they were not assembled in any way which is easy to make sense of. If I just give you a table of numbers that says, “Here’s the probability of this and here’s the probability of that. Here’s the side effects from this and here’s the side effects from that,” it’s very hard to just look at that and all of a sudden say, “Oh, so having looked at this piece of paper with all the numbers on it, I should do X.” Well, actually no.
What the model can do is say, “Look, here are some breakeven points. If your preferences are such that you really want to avoid certain kinds of side effects or you really want to avoid certain kinds of disabilities, then it’s likely that you’re going to fall into this area of the options curve versus a different part. Because when you get right down to what the actual number of decisions or options, I should say, for the patients, it’s often a yes/no. It’s often a “Do I have surgery? Do I not have surgery? Do I switch from the treatment I’m on to another treatment?” What the model’s very helpful for there, I think, is really sharpening the focus and saying, “I don’t need to know exactly what your preferences are, but if I know that you’re over on this half of the map, then it becomes easier to say what would be best for you.”
Howard Forman: We really appreciate the impact that you’ve had on public health and on society and really thankful that you continue to do this work. So thanks, Ed Kaplan, for joining us.
Harlan Krumholz: Thanks so much, Ed. Great to talk to you.
Edward Kaplan: I appreciate it. If I come up with a winner for the basketball tournament, I’ll make sure to let you know.
Harlan Krumholz: Yes. Just whisper it. Don’t tell everyone. Just whisper it in my ear.
Edward Kaplan: All right. Take care, everybody.
Harlan Krumholz: Thank you.
Edward Kaplan: Great to see you.
Harlan Krumholz: Thank you.
Edward Kaplan: Bye bye.
Howard Forman: Harlan, with all that is going on in the world, Senate confirmation hearings, the war in Ukraine, surging inflation, what is catching your attention right now?
Harlan Krumholz: Well, there certainly is a lot going on, and I’ll say that you wouldn’t think that a Supreme Court hearing in the Senate would be something compelling to watch, but actually, I have found it so interesting. To watch this individual, Judge Ketanji Brown Jackson, show such poise and to have been so accomplished and to see the wide range of questions that she’s facing, which really reflects the kind of split still in our country about what people think is important and what they want to bring up. But I can’t get away from the idea that this is just a remarkable moment in our history, to have a person like this about to ascend to the Supreme Court and to break barriers by doing it. It’s so long overdue, but as I do see the wars about Critical Race Theory and the tension and friction about it, I just can’t help to think that we need to get past this.
It just seems so strange to me that our country can’t really embrace the problems of our past. There’s so many people who just want to keep moving forward rather than really examine those lessons and then understand their echoes and implications for still things that are going on today. I told you, another thing to me that just struck me this week was, I know that you don’t follow football as closely as I do, but the Cleveland Browns have decided to pay I think something like $240 million to Deshaun Watson. Deshaun Watson’s a gifted quarterback, but who has been facing a lot of difficulties in Houston as many women came forward and accused him of sexual assault. Now a grand jury decided not to pursue charges, but he’s still under a lot of civil lawsuits, and he remains a very controversial figure around what exactly happened and how this happened.
I bear no ill will towards Mr. Watson. I’m just raising that despite all of that baggage, he was able to navigate a very large contract with Cleveland Browns who are willing to take on that risk. Compare that with a Super Bowl quarterback, Colin Kaepernick, who is essentially shunned from the NFL because he took a knee, because he took a stand for racism in this country and was trying to draw attention to issues around race in this country. For that act and for speaking out on behalf of people who have been victims of racism in the country, he was put in a position where he was unhirable within the NFL. No one would touch him. So it just shows you the miles we still need to go, that in some ways, a team would be willing to take the risk with someone who still has pending lawsuits around sexual assault and lots of questions around behavior.
Again, it may all work its way out, but I’m just saying it’s risk. It’s risk to do that, but it was too risky, too risky to hire a quarterback, a proven quarterback who had spoken out about race in this country. So it just shows me how far we have to go still, and it still makes me sad when I see that senators are raising such a ruckus about discussions of race within schools as if we really should pretend like none of this stuff exists. Some people say it’s more complicated than that, but for me, it seems pretty straightforward, and it’s a disappointment.
Howard Forman: Yeah. Look, this has been a momentous week with Judge Ketanji Brown Jackson speaking so eloquently, quoting the Reverend Dr. Martin Luther King. It brings me back to one of his famous quotes, which is, “The arc in the moral universe is long, but it bends towards justice.” In my lifetime, I’ve seen more justice, but we are far from being a just society.
Harlan Krumholz: I think when the history is told that this justice, Justice Ketanji Brown Jackson, will be one of the greats, really, when I see how articulate she is, how thoughtful she is, what her past record has been, I think she will be one of the greats.
You’ve been listening to Health & Veritas with Harlan Krumholz and Howie Forman.
Howard Forman: How did we do? To give us your feedback or to keep the conversation going, you can find us on Twitter.
Harlan Krumholz: I’m at H-M-K-Y-A-L-E. That’s hmkyale.
Howard Forman: And I’m @thehowie. That’s @T-H-E H-O-W-I-E.
Harlan Krumholz: Health & Veritas is produced with the Yale School of Management. Thanks to our researcher, Sherrie Wang, and to our producer, Miranda Shafer. Talk to you soon, Howie.
Howard Forman: Thanks, Harlan. Talk to you soon.