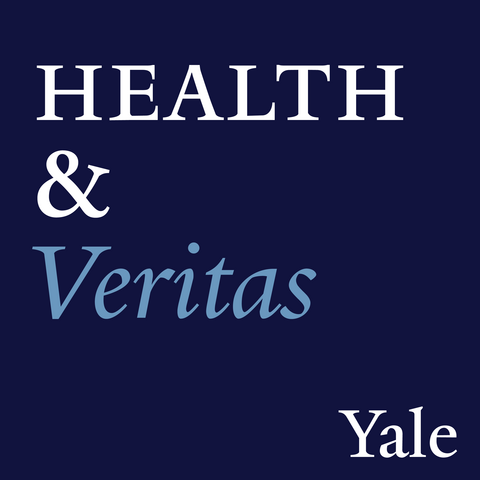
Dr. Rohan Khera: AI, Machine Learning, and Medicine
Subscribe to Health & Veritas on Apple Podcasts, Spotify, YouTube, or your favorite podcast player.
Howie and Harlan talk with Dr. Rohan Khera, a Yale cardiologist whose research interests include the use of advanced data science tools to improve diagnosis and treatment. They discuss how artificial intelligence and machine learning can supplement human expertise in summarizing current literature, interpreting electrocardiograms, and other areas.
Transcript
Harlan Krumholz: Welcome to Health & Veritas. I’m Harlan Krumholz.
Howard Forman: And I’m Howie Forman. We are physicians and professors at Yale. We’re trying to get closer to the truth about health and healthcare.
Harlan Krumholz: Today, on the cusp of Thanksgiving, the day before Thanksgiving, we’re going to be talking with Rohan Khera, a cardiologist who works on artificial intelligence and machine learning in medicine and is doing some real breakthrough research to, I think is going to help pave the way for a lot of new innovations. But first, Howie, we always start with something in health news that has your attention. So, let’s go. What’s got your attention this week?
Howard Forman: The number of people waiting for a psychiatric bed at our hospital and nationally is a big problem. I don’t see enough people talking about it. I talk to other physicians and healthcare people nationally, and they’re aware of it. I see it when I do my own rounds in the ER, which even though I’m a radiologist, I do rounds in the ER during each shift. And in my 25 years, I’ve never seen so many children, adolescents, and adults waiting a bed for mental health evaluation or treatment. I think this is just an under-discussed side effect of the pandemic. It’s not caused by COVID or the vaccines or the lockdowns as far as we can tell, because it’s occurring nationally, seemingly in large numbers everywhere, irrespective of phase of pandemic, phase of vaccinations, and so on.
And there’s no signs that it’s abating. We simultaneously have shortages of inpatient beds, so we cannot repurpose capacity easily. We’re having staffing problems separate from that, but it’s really the demand I’m talking about today. I just think the stress on all of us from the pandemic cannot be overstated. Those of us with supportive work, families, and friends are better protected. But even though we’ve done a great job, I think, of protecting people from physical illness and the economic effects of the pandemic, we’re not doing enough to address this epidemic of mental illness.
Harlan Krumholz: I couldn’t agree more.
Howard Forman: What about you, Harlan? What are you thinking about?
Harlan Krumholz: I thought I would maybe tout one of the papers that we did that came out this week, that focused on this question about how the pandemic has been affecting younger populations. There’s a widespread belief that really the only people at risk are those who are elderly and that the young are relatively spared and that is true with regard to risk, but young people are at low risk for a lot of things. What we wanted to do was look pretty closely at the leading causes of death among adults who were aged 25 to 44 and focus specifically on Texas, where there’s been a lot of controversy about how to protect people from the pandemic and whether there should be mass mandates, and also, there’s been some lagging stats on vaccination. So using records from the Texas Department of State Health Services, we looked at monthly mortality data and then also stratified it, looked at it separately by race and ethnicity, among Black, Hispanic, and White persons.
What we saw was that between March and December of 2020, as the pandemic really had entered into its first year, COVID-19 was a leading cause of death during the third quarter of 2020, and the second leading of cause of death during the fourth quarter. The implications for these young populations are quite profound. To see that it had risen to the number one cause of death in this age group makes it hard to ignore. Now again, for any single person, the risk was low, just like the risk of dying for someone in this age group is relatively uncommon. But if we start thinking about these leading cause[s] of death and how society reacts to them, how seriously we take them, it becomes hard to ignore. And in addition, what we see is a markedly disproportionate elevation and mortality among Hispanic residents. This was also something that was really showing this divergence between racial and ethnic groups. In Texas, these young Hispanic adults were the ones who were at the highest risk and the ones for whom the cause of death was really the most prominent.
Howard Forman: Look, I thought that paper was great. I saw that in JAMA Internal Medicine. Just want to highlight that you worked with our colleague Jeremy Faust on this. I do think it’s an important contribution into both the health equity literature, the COVID literature, and the intersection of those. It was great work.
So, Harlan, now’s the time for the main courses, so to speak, as we approach Thanksgiving. You’ve been great enough to invite Rohan Khera. I’ll turn it over to you.
Harlan Krumholz: He’s an assistant professor in the section of cardiology, and I wanted to have him on today because there’s so much going on around these words “artificial intelligence,” “machine learning,” “healthcare.” There’s such a, I think an exuberance about these terms and hopes and aspirations around them. But those of us who are also seeing patients know that the reality of our world is still very much like it was 30, 40 years ago. I mean, these remain promising innovations, but they have yet to really hit our day-to-day lives and the day-to-day lives of our patients. I was hoping that we could get Rohan to unlock for us, a little bit, what’s going on and what he sees as these sort of next and future steps, but let me start at a little earlier step.
He’s a graduate of a medical school in India, that’s the singular, best medical school in India. And the way you get in is simply by scoring at the top of a national test. It’s a test that anyone can take. And if you score at the very top, you can get in at no interviews, no essays. It’s just about test scores, and Rohan, we’ve spent a little bit of time here talking about medical schools and admission practices. First, I want to know how in the heck did you score so high that you got in? Then secondly, I just want to know, you’ve been here, you’ve been there. What do you think about that kind of system for admitting people to medical school?
Rohan Khera: I mean, the lack of interview shows like my personality issues, I would guess.
Harlan Krumholz: Not at all.
Rohan Khera: But I mean, in all fairness, I think it’s obviously equal parts luck and opportunity, and then an equal part like some effort. I would not discount the amount of time and effort it took on my part, on my family’s part, in ensuring that I had the opportunity to take those tests. And I think I spent a couple years of like dedicated preparation and did enough preparation that I was confident on that day that I would do well. On top of that, I was lucky that I could bring that into that test that day.
Harlan Krumholz: And just for calibration here, how many people take the test?
Rohan Khera: The year that I took it, about 80,000 individual people applied, about 50 get in.
Harlan Krumholz: We think so much, you’ve got to talk to people. You have to figure out who they are, what makes them tick, figure out if they’re going to be a good doctor. And I know this is true in China as well, you know, are countries where simply how you score, that’s going to depend on... What do you think about that?
Rohan Khera: No, I think it’s actually like, it has both ends of the thing. It actually allows people from disadvantaged backgrounds, so your ability of how you carry yourself, those reflect where you grew up, what kind of resources you had growing up. Those can be a disadvantage for individuals. I think that is a level playing field, that if you have the opportunity to study and you have the intellect, you’ll get through. That actually is the thematic basis for it. I think there is a challenge that people from advantaged backgrounds have better resources to even study. Even though that is designed to make a level playing field, it probably doesn’t as much. But because there are such large cultural differences, especially in India, I feel interviews can become a little challenging in that kind of environment.
I mean, yes, interpersonal skills are different, but our patient populations are so different. Every patient is so different socioeconomically, culturally, language-wise. There’s so many differences that are harder to appreciate. I think it does have an advantage, especially in our culture and environment, but having sort of, to your point, being here, I see the well-rounded individuals that come through the medical school path, especially the Yale School of Medicine and UT Southwestern and University of Iowa, for example, the three institutions that have had a longstanding relationship with med students. I’ve found that the most well-rounded students not only score well on their exams but were actually a good personality, did end up like having a lot of leadership potential. They actually ended up being leaders in their fields. I think that part may be missed by a test-centered thing, that you don’t actually recognize folks who have a broader understanding of medicine and the ability to take the field forward in a new way. I think that is a skill that the interview and the interpersonal aspect actually are missed in our testing.
Howard Forman: I would just say, Harlan and I have talked about this before on the podcast, and having sat on the admissions committee here for about 20 years up until a few years ago, it is so challenging. What you said summarizes it perfectly. It is all the more reason why we’re so lucky to have you today because you are the total package. I mean, just quantitatively, his scholarship, I don’t think I’ve ever seen somebody more productive in my entire career at this stage, by any measure, whether you look at someone’s first authorship or citations or whatever. I don’t think I’ve ever met somebody who’s made more substantive contributions than you have.
Rohan Khera: I mean, thanks for the generous comment.
Harlan Krumholz: Everybody’s hearing about all this fancy-schmancy stuff, mathematics and how data science is going to really rule the world. But can you share with the audience a little bit about maybe your global view of this? I mean, what’s going to happen in the next three to five years, and where do you think you’ll be 20 years from now with regard to the system? Will this have transformed everything or not?
Rohan Khera: I think for both good and bad, there is consistency in medicine. We tend to work the same way. So even though we’ve had the last decade, as you said before, of innovation, we have had very gradual intake on the actual clinical care pathways. For the next three to five years, I do feel we will see these under-the-hood innovations that actually will incorporate in our healthcare models, be it better screening models, be it better patient identification, avoiding patients falling through the cracks, things, Harlan, you and I have sort of talked about. Those will happen under the hood so that the clinicians’ day-to-day relationship with the patient does not change in any meaningful way, but we ensure that patients don’t have a bad outcome through safety events are prevented and we have better ways to screen individuals, better ways to detect disease, a better way to predict patients’ bad outcomes. I think those things will happen, to a lesser extent, but for certain we’ll start like seeing more and more of a digital health footprint.
Harlan Krumholz: But will it replace doctors? I mean, will this capacity make it different?
Rohan Khera: No. I personally think, you know, patients don’t come to us. Already, I feel the knowledge base grows at such a pace that a physician is unable to actually be the best arbitrator of knowledge or information. I mean, that’s already moved on to the web, you know, folks, even in our stage, you look at up-to-date for my own field, or like look at a few papers before I see a very complicated patient. I don’t think we will remain these—
Harlan Krumholz: And just for the audience, “up-to-date” as in electronic textbook. When I came through, you would actually have a real textbook, you’d find it, the day it came out it was out of date because it had come out. This “up to date” is something which you’re accessing online, where chapters could be revised within weeks of new studies, right?
Rohan Khera: Absolutely. And I think the key theme that I’m trying to say is that, the ability to actually think about amount of data is already exceeded by the human capacity, even for experts. The connection to the patients, the ability to actually meet them where they are, understand the unique social situation, their cultural situation, their medical problems, that diagnostic aspect of the medical problem may actually be augmented by these tools. But I suspect, vast majority of healthcare contact is not built around that. I think that’s irreplaceable. I mean, again, it may be augmented, same physician may be able to provide care not only in the U.S.—
Harlan Krumholz: You think that this is going to be more like autopilot. Like, we don’t go without a pilot, but that pilot’s augmented by tools.
Rohan Khera: Absolutely. And I think that’s a great analogy for the current-day position.
Howard Forman: I had an experience, two days ago, I think now, where I had to contact Delta Air Lines about a ticket I purchased two years ago, right before the pandemic. I was dreading that I knew I had to do this. Eventually, I was dreading it, I did it using their online, talking to a bot, basically. My entire time spent with them was a total of 10 minutes, and about two of those minutes, maybe less, was spent with the actual human being at the end. My refund was arranged. I’ve never had a better experience with that. The following day—
Harlan Krumholz: And we’re not sponsored by Delta, just for listeners.
Howard Forman: I want to make that clear. Yes. It was seamless. It was perfect. And it just made me realize the possibilities in medicine are at least as vast, if not more, than what Delta can achieve. And it benefits Delta, it benefits the consumer, so can it be in healthcare. I just wonder, having seen the type of work that you’ve worked on already is, do you see this as being consumer-centric and getting down to the consumer level at that level soon?
Rohan Khera: Absolutely. I think I can answer this question in two parts. One is the positive and one more for contemplative section. The first part is that, what you describe is natural language generation, where the machine has parsed enough text that it actually can generate text like a human. I think that’s AI in its form because the machine is acting like a human. That’s AI. The underlying aspect is, it’s interpretive. The natural language processing, the NLP aspect that you’ll see in literature and in like common parlance now, is actually interpreting your text for what it means and not just the word as written. So it’s not just a bag of words—do continually the words actually have a meaning associated with them? I think with enough information fed to algorithms that work on these, you can learn and generate something very similar in healthcare.
It already has happened, to a certain extent. And you can actually see, they’re all like smaller published studies of proof of concepts, but people have tried to do it in MyChart environment, have patients enter their complaints, what they’re feeling, and somebody would come back to you. There’s also some digital doctor startups that are trying to do early diagnosis of patients based on what they report. They’re all fairly, not as robust or as sensitive. I think that’s the second part of it. The ability to cancel a ticket may be ... the bar we have in medicine is so high for us, our ability to like lose something, a piece of information, a data point, or our patients. I think that sort of bar is what makes it more challenging, that we cannot have lost a heart attack patient. They were complaining about trouble swallowing, and we said, “Oh, you probably have reflux,” and it ended up being a heart attack. I think those kind of challenges about not having the breadth of, and actually not losing important pieces of information makes us more circumspect about such sort of strategies.
Harlan Krumholz: One of the things I think you’re doing that’s really interesting is taking information, in this case, from electrocardiogram, a signal, and maybe being able to see things that the human eye can’t pick up, that there may be information in the very raw form of the data as it’s collected that doesn’t manifest itself on the written tracing in a way that the human eye can pick up as being similar or different, so that we’ve created categories over time. Labels of what’s the same and what’s different based on what our eyes can perceive and what patterns we can describe, but you’re taking it to the next level, employing high-level mathematics on these signals, either from the paper tracing or from the actual raw signals themselves, in ways that might help us draw distinctions between people that we are interpreting as being similar, but actually the signal is suggesting may be different. Those differences may have implications for how people do or what treatments might be best for them. Maybe you can just say a little bit of how did you get onto that and what do you think about the future of that?
Rohan Khera: I think, Harlan, that’s an excellent area where machine learning and AI has the most promise, and it’s where we have high-frequency recordings or high-frequency data collection that we have summarized already. The printed ECG is already a summary of voltage recordings, with 12 leads in the heart. Actually, each voltage is recorded 300 times a second for a 300-Hertz recording, or more commonly like 600 times a second. As you can imagine, the degree of capture so that a single lead over 10 seconds at 600 Hertz has 6,000 data points, and you’ve got 6,000 data points times 12. So you’ve essentially constructed electrical map of the heart by checking the heart’s electrical activity in multiple directions. Conceptually, what we can say is that if there is a structural abnormality in the heart, it won’t only manifest as clinical disorders on the rhythm, but you would likely have aberrations in that electrical map of the heart.
I think that’s the conceptual model that I have sort of thought about. And again, it’s a growing field. Multiple investigations from different groups across the nation have found sort of these facts to be true. I think this is where some of the specific classes of models that have actually understood smaller signal patterns—and again, this is where machine learning really thrives—is the ability of pattern detection across the spectrum of data points that are high-frequency collections. I feel that allows us to sort of bring the electrocardiogram from the generation where we read stuff to a generation where we can actually deploy novel ways of thinking about new disease subtype subgroups in patient populations that we have to do advanced testing for.
Harlan Krumholz: Can you just define “machine learning” for folks? Just so everybody knows what you’re talking about.
Rohan Khera: Machine learning is a branch of analytics or mathematics where the machine doesn’t have to be explicitly taught how to learn patterns in the data. It sees the final product of what the data should look like. It actually iteratively goes through the data, finding what aspects of the data should be weighed how much to arrive at a label or an outcome that the machine is being told to learn. That’s the usual way of learning. And sometimes, the machine will, in what’s called “unsupervised learning,” where we don’t even tell [it] what it has to learn. The machine will just learn association between what co-localizes, what data points are together, what are farther apart. That’s the “unsupervised,” where the machine learns associations without explicitly telling it what it’s learning from the data.
Howard Forman: Can you just take that the next step and tell us two questions. One is, how do we distinguish artificial intelligence from machine learning? And two, what percent—in any way, you don’t have to use an actual number—of what we’re taught about really fits into just machine learning versus what might be described as artificial intelligence?
Rohan Khera: But I would say artificial intelligence is this like broad field that where the machine mimics human behavior and machine learning is a subset within artificial intelligence where the machine is the math part, where it actually learns signals and interprets the data, in a way. And in a way, I would say AI applications end up using machine learning, but there may be non-machine-learning aspects to it too. But for the most like day-to-day use, I would say now they’ve kind of come together for the most part where ML drives AI applications, “ML” being machine learning, and “AI” being artificial intelligence.
Harlan Krumholz: You’re a data scientist, and you’re also a clinician. Can you just reflect on what you think are going to be, or are already, the points of resistance? What is it that makes it hard for these novel new approaches to be put into practice? And what is it going to take for them to be worthy of being integrated into practice and then having the medical establishment tack toward them? Because I can even imagine in the beginning of sort of autopilot and instrumentation and pilots, there were some pilots who were sort of, “Hey, I don’t need those instruments. I know how to fly.” And maybe it took a while then also for that to be transformed. But I see a lot of resistance in medicine where people are suspicious of these new methods. What do you think are going to be points of friction and how we’re going to get around them or through them?
Rohan Khera: I think that’s an excellent point. I think we have been dogmatic in medicine for a while. With the point, idea being that we will protect our patients from technology that has not been fully developed or fully scoped out; it comes the same way from drug and device companies where somebody promoted a product too aggressively. And we were protecting our patients from that sort of like aggressive marketing. What we have to sort of think about these technologies are, is in the same light. Clinicians trust these technologies. If, one, they can understand what happens, and it doesn’t actually have to be the math. It has to be that it actually was the data that were used inside to develop these models were actually accurate. And the labels we used were right; there’s the right population. Say for an example of the heart attack, we actually found the patients who actually had a heart attack when the model learned.
Two, I think the key aspect is that it does not, I think, especially in this day and age where we have a keen understanding of bias, where we understand that the data were not derived in a very homogenous population that does not generalize to our patients, say at the Yale New Haven Hospital, more diverse and more challenging populations. I think to that effect, from pure model development, or like learning about these new technologies, there is a general technology push towards interpretability, so actually adding a layer where you as a clinician or as a human being can see what components of the data. Say for the example, the electrocardiogram; you can now say which pixels or which voltage areas did the model learn on, and you could do it for the things that you can visually diagnose, like some of the clinical disorders and say, if the model can do that in an appropriate manner and it not learn like a heuristic or learn like a shortcut to the data, then you would engender trust about things that the humans cannot interpret.
So I feel that would be a key aspect, more interpretability, broader access to the data streams that actually go in. And finally, I think, the third part, I think it’s just like drugs and devices, randomized clinical trials, or large clinical studies that prove that these were actually generalized and have modified care or improved care in a real meaningful way.
Harlan Krumholz: I think if we can show it’s a competitive advantage, if the people who are using these tools, just like the pilots who were using these tools would [be] safer, like obviously the pilots had a lot more at stake because, the plane goes down, they go down! But we often say that the consequence for the doctors that they have to feel that their success is tied into this.
Rohan Khera: Absolutely. I think that’s true.
Howard Forman: Do you think that where your priorities are, the things that you see as the lowest-hanging fruit and perhaps the most successful ways in which AI, machine learning can be applied right now, are they being commercialized at the right rate? Are there areas that you think somebody listening to this show should be thinking about more from the point of view, because you’re doing a lot of the scholarship that feeds into this. But one of the concerns I have is that a lot of the commercialization has been all about productivity and not necessarily about improving ultimate outcomes or expanding knowledge. I’m just wondering what your thoughts are on that.
Rohan Khera: I mean, again, that’s an excellent question because it comes up often, especially in our group discussions too. I would say, I think it is changing a lot that although the initial miles were like tech- and data-centric that would offer you a faster machine, a better-aligned electronic health record, the novel sort of way of developing models and bringing them to market through academically startups or academic entrepreneurial collaborations is actually on the rise. I mean, it’s the case for many members of our community who essentially see an up.... I think there’s like always this bar, right? You have to develop it enough that when you take it to the commercial grid, you can actually trust it and actually get the investment you need to push it forward. But also not do it too late, so that folks who don’t understand technology, who haven’t developed it, have an inferior product that takes away the trust that people need to develop in these sort of tools. I think it’s a balance, but I think in my sort of sense of the world, this is one area where clinician[s] and data scientists are taking charge in commercializing and finding ways to commercialize their own sort of tools.
Harlan Krumholz: A question I have for you then is, what’s going to be the role of academia? Academic institutions, given that there’s so many of these businesses with such deep pockets and so many data scientists, where’s the niche going to be for academics to contribute to this? What is their role? How do you see that?
Rohan Khera: I think, Harlan, that’s the most important point there. I feel like, especially your background, my background. I mean, we understand basic challenges with learning from healthcare data and how complex it is and building studies, learning from the data and technology. It’s more than just the model aspect of it. It is actually the design of the study, how we learn from these data and how we go ahead with it. So I feel the academics have the role to understand that these are just tools in the broad scheme of learning, causal inference learning about what impacts care truly, rather than just to shortcut on a data end. To the second part, I think the academic model has to evolve as we actually get to a point where these things need more investment, they need to be rapid-cycle. Our current grant funding mechanisms are slower, don’t allow innovation to happen at that scale or at pace. I think that’s the bigger challenge that I see from an academic standpoint, that academics would find the environment to be restrictive and would move on to other opportunities so they can actually bring about the impact that they sought to achieve as their academic role anyways.
Harlan Krumholz: I’ll just add one other quick thing on the academic side, which is that I believe that the privilege that we have and the responsibility we have is that we’re proponents for the best interest of society. We don’t have stockholders. We don’t have other interests to which we have allegiance or allegiances to try to push forward knowledge that will help society. In the end, that gives us a certain amount of freedom to promote those interests and pursue research that we think will help people. Sometimes that can then end up being commercialized. Sometimes it can end up just being given away. There’s lots of ways that that may disseminate, but we’re not constrained by the need to hit a mark the next quarter or to have investors say to us, “What have you done for us lately for us to be able to give up on that plan?,” because somebody has some short-term needs that are demanding, that we turned in the way. If we have come up with a good idea also, Rohan, I mean, in academia, we can pursue it if we can get some support for it, or we can just spend our own time on it. I continue to think that’s a privilege, plus the opportunity to work with students who renew us every day. That’s a big plus. Anyway, that’s my plug for academia. I don’t know. I felt the need to kind of throw that in.
Howard Forman: No, that’s a great way to go out. And in the spirit of Thanksgiving, thank you very much.
Harlan Krumholz: Thanks, Rohan.
Rohan Khera: Thank you so much. Thanks for having me.
Howard Forman: In the spirit of Thanksgiving, Harlan, what is one thing to be thankful for? I’ll make it easy for you and exclude me from your options.
Harlan Krumholz: I would definitely be thankful for you, you know that. I try to express that to you. Look, let me change the question a little bit. As we move on to Thanksgiving, how am I thinking about it? I’m thinking that, we’ve got to double down on what we’re really grateful for. We are still in the midst of turbulent times. And I was saying to my wife the other day that gosh, we’re just six months from being two years into—or, less than six months—we’re just a few months away from being two years into this pandemic without really an end in sight and uncertainty about how this is all going to play out. We’re trying to do the best job we can to generate knowledge, get smarter, be able to handle it better. But so many of us are just tired of it.
Yet it’s still persisting and posing a threat, the issues around politics and race in this country and a whole wide range of other issues, the economic issues. I think the way we get through this is not only to work hard to try to make the world better every day the best we can, but within the smaller aspects of our life to really recognize what we are grateful for. What really helps make us happy and brings joy, and for which we can’t take for granted.
I guess for me, that’s around family and friends, relationships, opportunities to do good things with others. I think that’s part of the way that we get through this, that we maintain our own sanity, is we do realize that there are always things that we can be grateful for. And we need to double down on that a bit, because it’s easy to get in a hole of feeling like “woe is us,” because there’s a lot going on for sure. And a lot of uncertainty, but there’s also a lot that we can be thankful for. As we come into this Thanksgiving, that’s what I’m doing. I’m thinking hard about all the things that I’m grateful for. And I’m going to try to work hard to make the world better too, at the same time.
Howard Forman: I agree with you, and I can just tell you how much I appreciate you because you do make the world a better place. I’ll just add to that and say, in addition to friends and family, life and health, I would offer that this year has been testing both our individual and collective resiliency, as I mentioned at the top of the podcast. I’m so thankful for the many people who are willing to take up this challenge on this day and every day to make our country a safer and more equitable place. I believe that this transcends party, it transcends ideology, passionate individuals advocating for those who are less fortunate, if you don’t have a voice and/or are marginalized or minoritized. Those are the individuals that are making a real difference, and I am sincerely grateful and thankful for them.
Harlan Krumholz: Amen to that. That’s really beautifully said, and we should all be thankful for the people who put in the sacrifices and are working hard on behalf of others. That’s really great, really great, Howie. You’ve been listening to Health & Veritas with Harlan Krumholz and Howie Forman.
Howard Forman: How did we do? To give us your feedback or to keep the conversation going, you can find us on Twitter.
Harlan Krumholz: I’m at H-M-K-Y-A-L-E, hmkyale.
Howard Forman: And I’m at the Howie. That’s at T-H-E-H-O-W-I-E.
Harlan Krumholz: Health & Veritas is produced with the Yale School of Management. Thanks to our researcher, Sherrie Wang, and to our producer, Blake Eskin of Noun & Verb Rodeo. Talk to you soon, Howie. Have a nice Thanksgiving.
Howard Forman: Thanks, Harlan. Happy Thanksgiving to you and everyone. Take care.