How Big Investors Avoid Market Predators and Keep Trading Costs Low
Researchers have generally believed that as large institutional investors make bigger trades, their trading costs rise accordingly. Research from Yale SOM’s Tobias Moskowitz, making use of industry data, examines the strategies of large-scale traders and finds that they take a slow-and-steady approach to keep costs down and outsmart market predators.
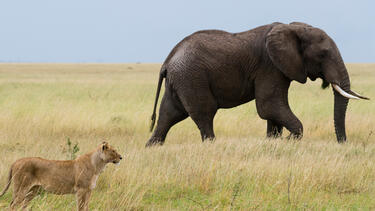
Patience is a virtue—and for large institutional investors, it’s a profitable one.
A paper co-authored by Tobias Moskowitz of Yale SOM reveals that large traders such as investment banks, mutual funds, and hedge funds minimize the costs of trading by adopting a slow-and-steady investment strategy. Their actual costs, the research revealed, are significantly lower than previous estimates by other scholars. Moskowitz, and his co-authors, Andrea Frazzini and Ronen Israel of AQR Capital Management, were also able to compare how trading costs vary across global markets with different rules.
The paper offers “a unique look into what institutional costs really look like, and…they look quite different than other estimates from the past,” says Moskowitz.
Trading costs consist of all of the expenses that institutions incur while trying to buy and sell stocks. These include commissions and fees as well as fluctuations in price that occur in the midst of buying and selling. Financial scholars have long speculated about the trading costs of large institutions, who are tight-lipped about their inner workings. But understanding how institutional traders operate is important, because these entities play a key role in the healthy functioning of financial markets.
To understand how large institutions control their costs, the researchers turned to AQR Capital Management, a global investment management firm where Moskowitz is a principal. AQR provided behind-the-scenes data from $1.7 trillion in trades across 21 global markets over a 19-year period. Moskowitz, Frazzini, and Israel also used publicly available information from other large institutions to supplement and confirm their findings.
Like many of its peers, AQR uses specialized software to execute trades. Researchers and portfolio managers decide what to trade—which stocks and how many shares—but an algorithm decides how to achieve that goal in the cheapest way possible, all monitored by human traders.
The biggest single factor influencing trading costs is what’s called price impact—that is, the effect of a transaction itself on stock price. Individual investors, who buy and sell small numbers of stocks at a time, don’t typically have much ability to influence price (the recent GameStop surge notwithstanding), but large institutions, who may be dealing with millions of shares at a time, do.
Say you’re a large institution seeking to buy a million shares of a particular company. If the stock is highly liquid (meaning, traded frequently and easily available) your purchase may not affect the price very much. But for an illiquid stock, it may take time for a market-maker—the person who matches buyers and sellers, taking a small cut in the process—to find those million shares. To entice sellers, the market-maker will have to boost the price of the stock. For large institutions, the fluctuations in price that occur over the course of a transaction can significantly increase their costs.
In the last 15 years, yet another challenge has emerged: high-frequency traders, who use complex computer algorithms to detect when other traders are buying or selling many shares of a stock. Such moves trigger the algorithm to swoop in within microseconds and bid the price up or down.
So how do large institutions like AQR avoid escalating prices and the prying eyes of high-frequency traders? Patience. Rather than buying a million shares all at once, their software takes a particular goal—say, buy a million shares of stock in a particular company—and breaks it down into a series of smaller, less detectable transactions over a longer period of time
“If you have to trade immediately, you’re going to pay a high price. If you don’t reveal that you need to trade all this stuff by the end of the week, that makes you hard for predators to detect.”
The researchers’ unique access to AQR’s data meant “we actually know the intent of the trade and can look at the whole trade broken up into its pieces,” Moskowitz explains. Rather than viewing each smaller trade as its own transaction, they understood the larger goal and calculated trading costs accordingly.
AQR’s cost-minimizing strategy also relies heavily on what are called limit orders—that is, an order to buy or sell only if the stock reaches a certain price. If the stock doesn’t reach the limit price in the right time period, the algorithm cancels the limit order. The downside is that a limit order may not be filled; the upside, of course, is that sitting tight prevents overpaying.
Using these means, the software covers its tracks, allowing large institutions to minimize price impact, outsmart high-frequency traders, and keep their trading costs down.
“If you have to trade immediately, you’re going to pay a high price,” explains Moskowitz. But if you have the option, “[you can] be patient, and what that means is don’t reveal that you need to trade all this stuff by the end of the week.… That makes you hard for predators to detect, and that’s what most institutions do.”
Many financial scholars had suspected that price impact was likely the most significant contributor to institutions’ trading costs. Some believed that trading costs always increased as the number of shares being bought or sold grew.
That’s true up to a point, the researchers found. The size of the trade is, indeed, the biggest predictor of trading costs. The more shares you try to buy or sell at once, the more you’ll pay.
But in practice, thanks to institutions’ careful maneuvering to limit price impact, the relationship between trade size and trade cost is not linear, as some believed. As the size of the trade goes up, trading costs “do increase—but they increase at a decreasing rate,” following a concave curve, Moskowitz explains. The discovery that trade size and cost aren’t linearly related helps to explain why trading costs are so much lower than previously thought.
The data also allowed the researchers to compare how real-world trading costs differ across markets. Average trading costs are lower on the New York Stock Exchange than Nasdaq and international markets, they found. In addition, markets that allow companies to trade on multiple exchanges tend to have lower average trading costs—not surprising, in that buyers and sellers can seek out the exchange offering the best price. While the quoted prices may vary only a fraction of a cent, the difference adds up.
Ultimately, low trading costs benefit everyone, Moskowitz argues. If the costs are too high, investors won’t trade, and prices won’t accurately reflect everything we know about a particular company at a given time. But when trading costs are low, investors can act quickly on new information, resulting in “the right allocation of resources,” Moskowitz explains—strong companies thrive and weaker ones flounder. For the good of all of us, “you want capital to flow where society wants it to go.”